Streamlining MongoDB Analytics with AWS
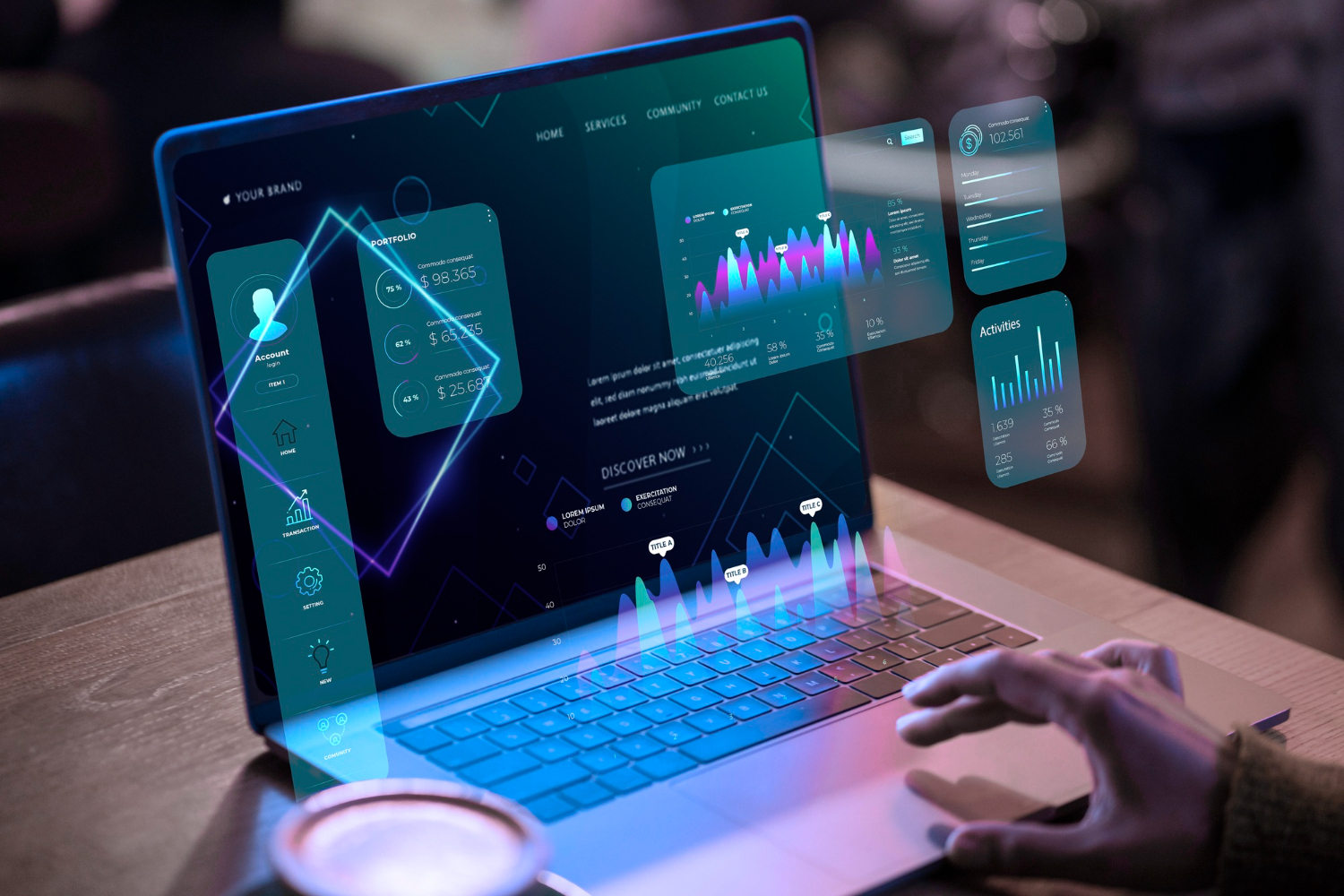
Key Challenges
Unosecur is facing difficulties in efficiently tracking customer activities and accessing insights due to the complex and time-consuming nature of querying MongoDB data. This manual process hinders decision-making and requires technical expertise for data retrieval and analysis.
Key Results
These key results demonstrate how the solution implemented by Ankercloud on AWS significantly enhanced Unosecur's data management capabilities, from efficient data processing and enhanced accessibility to improved data quality and automation, ultimately supporting informed decision-making and operational efficiency.
Overview
Unosecur manages data on MongoDB and requires timely insights and analytics, which are currently obtained through complex queries. Customers requested a centralized, easy-to-access platform for insights, including incremental data updates, to streamline decision-making and save time.
Challenges
- Inefficient Data Tracking:
- Issue: Difficulty in efficiently tracking customer activities and accessing insights due to the manual and complex nature of querying MongoDB data.
- Impact: This inefficiency hinders timely decision-making and operational agility, as insights are not readily available and require significant technical expertise and time for data retrieval and analysis.
- Complex Querying Process:
- Issue: The current process of querying MongoDB for insights is complex and time-consuming.
- Impact: It results in delays in obtaining critical business insights, which are essential for making informed decisions. The complexity also limits accessibility to insights, as it requires specialized knowledge and skills to navigate and extract data effectively.
- Lack of Centralized Insights:
- Issue: Insights and analytics are scattered and not centralized, making it challenging for stakeholders to access and collaborate on critical data.
- Impact: Decision-makers face difficulty in accessing a unified view of data insights, hindering collaboration and potentially leading to fragmented decision-making processes.
- Manual Data Retrieval and Analysis:
- Issue: Manual processes for data retrieval and analysis require significant human effort and are prone to errors.
- Impact: The reliance on manual processes increases the risk of errors and inconsistencies in data analysis, impacting the reliability and accuracy of business insights derived from MongoDB data.
- Demand for Incremental Updates:
- Issue: Customers require incremental updates to data insights, which are currently not efficiently managed or integrated into existing data sets.
- Impact: Lack of incremental updates limits the ability to provide real-time insights and hampers responsiveness to changing business needs and market conditions.
These challenges highlight the complexities and inefficiencies in Unosecur's current data management and analytics processes, underscoring the need for a streamlined and automated solution to enhance operational efficiency and decision-making capabilities.
Solution
Infrastructure: Hosted entirely on AWS. Steps Taken:
- Data Lake and Data Warehouse Setup: Created a Data Lake in AWS S3 to automatically import data daily from MongoDB using AWS Glue jobs. Established separate Glue jobs for ETL processes to transform and load data into the Data Lake.
- Redshift Data Warehouse: Implemented an Amazon Redshift cluster to create a Data Warehouse for structured data storage and analysis. Used Redshift's COPY command to load transformed data from S3 into Redshift for querying.
- Visualization: Deployed Metabase on an AWS EC2 instance for data visualization. Established a connection between Redshift and Metabase to ensure real-time data updates and visualization.
- Production Deployment: Tested and replicated the setup from development to production environments.
- Monitoring and Alerting: Created AWS CloudWatch dashboards and alerts to monitor the health and performance of the Redshift cluster.
Business Outcome
- Efficiency Gains: Centralized data extraction and loading into S3 improved efficiency and reduced manual effort, potentially leading to cost savings.
- Enhanced Data Accessibility: Data Lake and Data Warehouse setup facilitated easy access to data for analytics, enabling quick and informed decision-making.
- Improved Data Quality: ETL operations allowed for data transformation and filtering, enhancing data relevance and quality for analysis.
- Automation Benefits: Automated daily data imports ensured up-to-date information availability without human intervention, boosting operational efficiency and reducing errors.
- Monitoring and Alerting Benefits: CloudWatch monitoring and alerts enhanced proactive management of Redshift cluster health, minimizing downtime and ensuring continuous data availability for analysis. This categorization helps clarify the challenges faced by Unosecur, the steps taken to address them, and the positive business outcomes achieved through the AWS-based solution implemented by Ankercloud.