Resources
The latest industry news, interviews, technologies and resources.
Enhancing DDoS Protection with Extended IP Block Duration Using AWS WAF Rate-Based Rules
Problem
DDoS attackers use the same IPs to send many HTTP requests once the AWS WAF rate limit rule removes the block. The default block lasts only for a definite time, so attacks repeat again. We need a solution that makes the block time for harmful IPs last indefinitely, keeping them blocked until the attack persists.
Solution Workflow
- CloudFormation: Use the predefined CFT template to set custom block time for harmful IPs. Adjust by how severe the attack is.
- EventBridge & Lambda: Let EventBridge call a Lambda function every minute. The function checks AWS WAF’s rate rule for blocked IPs.
- Store in S3: Save blocked IPs in an S3 bucket with timestamps for records.
- Update WAF Custom IP Sets: Lambda revises WAF custom IP sets by keeping IPs within block time. It also drops IPs that passed the block period.
- Regular Updates: Run the process every minute to keep only harmful IPs blocked and avoid an outdated, heavy block list.
Deploying the Solution
- Download the CloudFormation Template:
Download the customized AWS CloudFormation template (customized-block-period-template.yaml) from the solution’s GitHub repository. - Create a Stack in CloudFormation Console:
Open the AWS CloudFormation console, then create a new stack with the downloaded template. Check the CloudFormation User Guide for detailed instructions for stack creation.
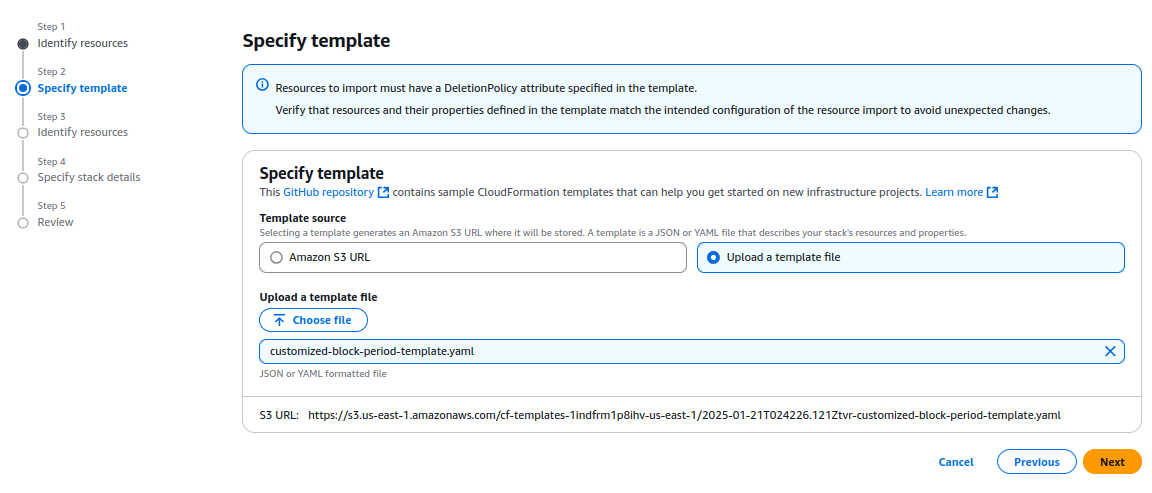
- Specify Stack Details:
On the Specify Stack Details page, type a unique stack name. Enter the required parameters, such as blocking duration and configuration settings listed in the prerequisites.

- Provisioning Resources:
The template provisions several AWS resources, including:
- AWS WAF IP Sets, which store the blocked IPs.
- An Amazon EventBridge Rule that triggers the Lambda function at regular intervals.
- Amazon S3 Buckets to store the blocked IP addresses and their timestamps.
- AWS IAM Roles with permissions to allow Lambda functions to query AWS WAF and access other required resources.
- The AWS Lambda function itself, which performs the logic for tracking and updating the blocked IP addresses.
- Deploy and Apply the WAF Rule:
Deployment takes under 15 minutes. When the stack shows CREATE_COMPLETE, build a custom AWS WAF rule to apply custom IP sets and block the malicious IPs.
%20(1).png)
6. Reviewing IPs that are Blocked:
Go to the IP Sets section on the AWS WAF console. Choose the set named with the prefix "IPv4-IPset." You can check the list of IPs blocked by the rate limit rule in the set produced by the stack.
7. Whitelisting or Removing Specific IPs from the Blocked List
To remove an IP from the blocked list, merely deleting it from the IP set in the AWS WAF console does not work. This is because the IP set updates every minute with a JSON file stored in an S3 bucket (controlled by the CloudFormation template).
To remove an IP properly, delete it from the JSON file; then upload the revised file to the S3 bucket. You may use a Lambda script to automate this process. The script lets you choose the IP to remove; it completes each required step.
You can find the environment variable details and the Python code for the script here:

Blocking Requests Originating from Referrer URLs
Problem Statement:
Third-party websites might copy images or content from your site and use them on their platforms. These requests come via referrer URLs.
Solution:
To block such requests, follow these steps:
- Identify the Referrer URL:
- Open the site suspected of scraping your content in a browser.
- Right-click on the page and select Inspect to open the developer tools.
- Navigate to the Network tab and reload the page.
- Look for requests made to your site. For example, if the site https://www.webpagetest.org/ is scraping your images, you might find requests to your domain in the list.
- Identify the image being used (e.g., twitter.svg), and click on the request.
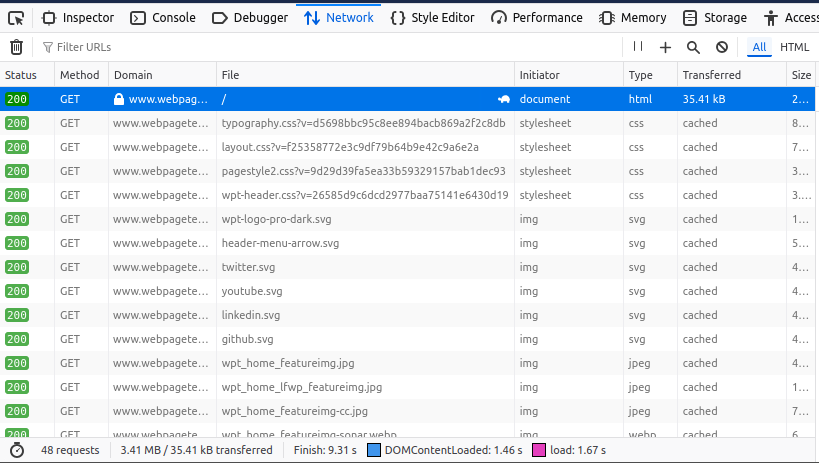
- Retrieve the Referrer URL:
- In the request details on the right panel, locate the Headers section.
- Scroll to find the Referer value. This will show the URL of the site making the request (e.g., https://www.webpagetest.org/).
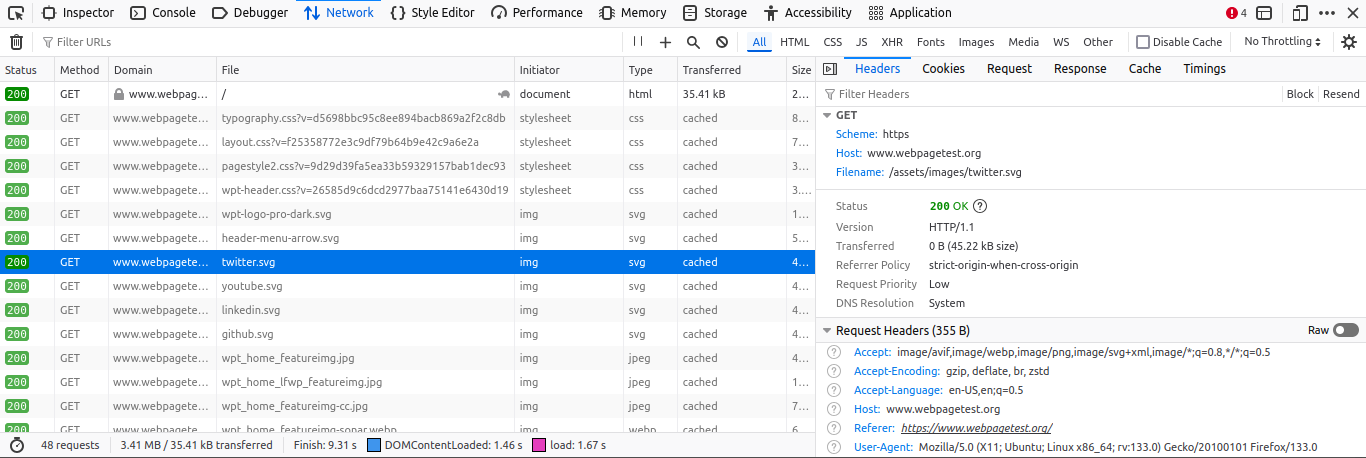
- Block the Referrer in AWS WAF:
- Open the AWS WAF console and create a new Custom Rule.
- Set the Inspect field to Single Header.
- Use Referer as the Header Field Name.
- Set Match Type to Exactly matches string.
- Enter the referrer URL (e.g., https://www.webpagetest.org/) in the String to Match field.
- Set the Action to Block. You can optionally configure a custom response code for blocked requests.
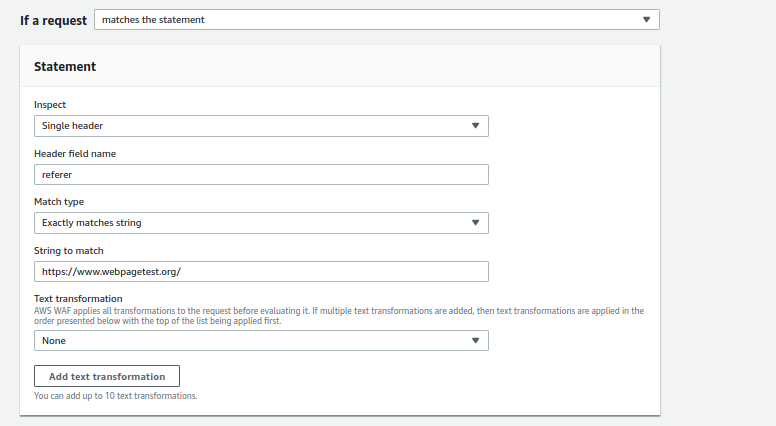
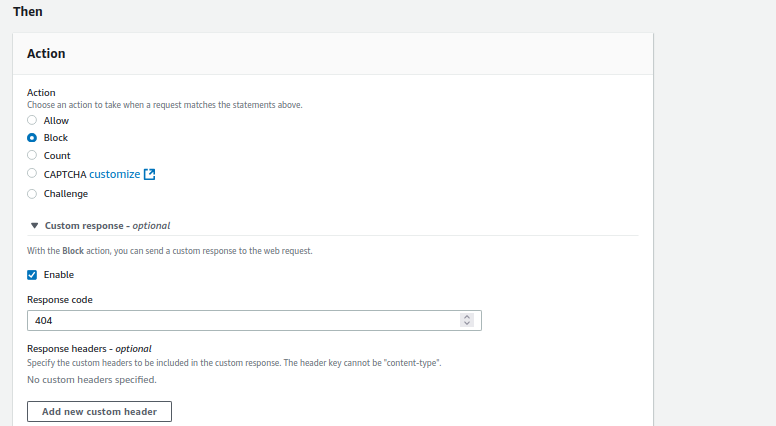
Outcome
By enforcing this rule, you can block requests from specific referrer URLs stopping site mirroring and web scraping by third-party sites.
Automating AWS Amplify: Streamlining CI/CD with Shell & Expect Scripts
Introduction
Automating cloud infrastructure and deployments is a crucial aspect of DevOps. AWS Amplify provides a powerful framework for developing and deploying full-stack applications. However, initializing and managing an Amplify app manually can be time-consuming, especially when integrating it into a CI/CD pipeline like Jenkins.
This blog explores how we automated the Amplify app creation process in headless mode using shell scripting and Expect scripts, eliminating interactive prompts to streamline our pipeline.
Setting Up AWS and Amplify CLI
1. Configure AWS Credentials
Before initializing an Amplify app, configure AWS CLI with your Access Key and Secret Key:
aws configure
2. Install and Configure Amplify CLI
To install Amplify CLI and configure it:
npm install -g @aws-amplify/cli
amplify configure
This will prompt you to create an IAM user and set up authentication.
Automating Amplify App Creation
1. Initialize the Amplify App Using a Script
We created a shell script amplify-init.sh to automate the initialization process.
amplify-init.sh
#!/bin/bash
set -e
IFS='|'
AMPLIFY_NAME=amplifyapp
API_FOLDER_NAME=amplifyapp
BACKEND_ENV_NAME=staging
AWS_PROFILE=default
REGION=us-east-1
AWSCLOUDFORMATIONCONFIG="{\
\"configLevel\":\"project\",\
\"useProfile\":true,\
\"profileName\":\"${AWS_PROFILE}\",\
\"region\":\"${REGION}\"\
}"
AMPLIFY="{\
\"projectName\":\"${AMPLIFY_NAME}\",\
\"envName\":\"${BACKEND_ENV_NAME}\",\
\"defaultEditor\":\"Visual Studio Code\"\
}"
amplify init --amplify $AMPLIFY --providers $AWSCLOUDFORMATIONCONFIG --yes
Run the script:
./amplify-init.sh
2. Automating API and Storage Integration
Since Amplify prompts users for inputs, we used Expect scripts to automate API and storage creation.
add-api-response.exp
#!/usr/bin/expect
spawn ./add-api.sh
expect "? Please select from one of the below mentioned services:\r"
send -- "GraphQL\r"
expect eof
add-storage-response.exp
#!/usr/bin/expect
spawn ./add-storage.sh
expect "? Select from one of the below mentioned services:\r"
send -- "Content\r"
expect eof
These scripts eliminate manual input, making Amplify API and storage additions fully automated.
Automating Schema Updates
One of the biggest challenges was automating schema.graphql updates without manual intervention. The usual approach required engineers to manually upload the file, leading to potential errors.
To solve this, we automated the process with an Amplify Pull script.
amplify-pull.sh
#!/bin/bash
set -e
IFS='|'
AMPLIFY_NAME=amp3
API_FOLDER_NAME=amp3
BACKEND_ENV_NAME=prod
AWS_PROFILE=default
REGION=us-east-1
APP_ID=dzvchzih477u2
AWSCLOUDFORMATIONCONFIG="{\
\"configLevel\":\"project\",\
\"useProfile\":true,\
\"profileName\":\"${AWS_PROFILE}\",\
\"region\":\"${REGION}\"\
}"
AMPLIFY="{\
\"projectName\":\"${AMPLIFY_NAME}\",\
\"appId\":\"${APP_ID}\",\
\"envName\":\"${BACKEND_ENV_NAME}\",\
\"defaultEditor\":\"code\"\
}"
amplify pull --amplify $AMPLIFY --providers $AWSCLOUDFORMATIONCONFIG --yes
This script ensures that the latest schema changes are pulled and updated in the pipeline automatically.
Integrating with Jenkins
Since this automation was integrated with a Jenkins pipeline, we enabled "This project is parameterized" to allow file uploads directly into the workspace.
- Upload the schema.graphql file via Jenkins UI.
- The script pulls the latest changes and updates Amplify automatically.
This method eliminates manual intervention, ensuring consistency in schema updates across multiple environments.
Conclusion
By automating AWS Amplify workflows with shell scripting and Expect scripts, we achieved: Fully automated Amplify app creation
Eliminated manual schema updates
Seamless integration with Jenkins pipelines
Faster deployments with reduced errors
This approach significantly minimized manual effort, ensuring that updates were streamlined and efficient. If you're using Amplify for your projects, automation like this can save countless hours and improve developer productivity.
Have questions or feedback? Drop a comment below!
Configuring GKE Ingress: Traffic Routing, Security, and Load Balancing
GKE Ingress acts as a bridge between external users and your Kubernetes services. It allows you to define rules for routing traffic based on hostnames and URL paths, enabling you to direct requests to different backend services seamlessly.
A single GKE Ingress controller routes traffic to multiple services by identifying the target backend based on hostname and URL paths. It supports multiple certificates for different domains.
FrontendConfig enables automatic redirection from HTTP to HTTPS, ensuring encrypted communication between the web browser and the Ingress.
BackendConfig that allows you to configure advanced settings for backend services. It provides additional options beyond standard service configurations, enabling better control over traffic handling, security, and load balancing behavior.
Setup GKE ingress with application loadbalancer
To specify an Ingress class, you must use the kubernetes.io/ingress.class annotation.The “gce” class deploys an external Application Load Balancer
apiVersion: networking.k8s.io/v1
kind: Ingress
metadata:
name: my-ingress
annotations:
kubernetes.io/ingress.class: “gce”
Configure FrontendConfiguration:
apiVersion: networking.gke.io/v1beta1
kind: FrontendConfig
metadata:
name: my-frontend-config
spec:
redirectToHttps:
enabled: true
The FrontendConfig resource in GKE enables automatic redirection from HTTP to HTTPS, ensuring secure communication between clients and services.
Associating FrontendConfig with your Ingress
You can associate a FrontendConfig with an Ingress. Use the “networking.gke.io/v1beta1.FrontendConfig” to annotate with the ingress.
apiVersion: networking.k8s.io/v1
kind: Ingress
metadata:
annotations:
networking.gke.io/v1beta1.FrontendConfig: “my-frontend-config”
Configure Backend Configuration:
apiVersion: cloud.google.com/v1
kind: BackendConfig
metadata:
name: my-backendconfig
spec:
timeoutSec: 40
BackendConfig to set a backend service timeout period in seconds.The following BackendConfig manifest specifies a timeout of 40 seconds.
Associate the backend configuration with service:
apiVersion: v1
kind: Service
metadata:
annotations:
cloud.google.com/backend-config: ‘{“ports”:{“my-backendconfig”}}’
cloud.google.com/neg: ‘{“ingress”: true}’
spec:
ports:
- name: app
port: 80
protocol: TCP
targetPort: 50000
We can specify a custom BackendConfig for one or more ports using a key that matches the port’s name or number. The Ingress controller uses the specific BackendConfig when it creates a load balancer backend service for a referenced Service port.
Creating an Ingress with a Google-Managed SSL Certificate
To set up a Google-managed SSL certificate and link it to an Ingress, follow these steps:
- Create a ManagedCertificate resource in the same namespace as the Ingress.
- Associate the ManagedCertificate with the Ingress by adding the annotation networking.gke.io/managed-certificates to the Ingress resource.
apiVersion: networking.gke.io/v1
kind: ManagedCertificate
metadata:
name: managed-cert
spec:
domains:
- hello.example.com
- world.example.com
Associate the SSL with Ingress
apiVersion: networking.k8s.io/v1
kind: Ingress
metadata:
name: ingress
annotations:
networking.gke.io/v1beta1.FrontendConfig: “my-frontend-config”
networking.gke.io/managed-certificates: managed-cert
kubernetes.io/ingress.class: “gce”
associate it with the managed-certificate by adding an annotation.
Assign Static IP to Ingress
When hosting a web server on a domain, the application’s external IP address should be static to ensure it remains unchanged.
By default, GKE assigns ephemeral external IP addresses for HTTP applications exposed via an Ingress. However, these addresses can change over time. If you intend to run your application long-term, it is essential to use a static external IP address for stability.
Create a global static ip from gcp console with specific name eg: web-static-ip and associate it with ingress by adding the global-static-ip-name annotation.
apiVersion: networking.k8s.io/v1
kind: Ingress
metadata:
name: ingress
annotations:
networking.gke.io/v1beta1.FrontendConfig: “my-frontend-config”
networking.gke.io/managed-certificates: managed-cert
kubernetes.io/ingress.class: “gce”
kubernetes.io/ingress.global-static-ip-name: “web-static-ip”
Google Cloud Armor Ingress security policy
Google Cloud Armor security policies safeguard your load-balanced applications against web-based attacks. Once configured, a security policy can be referenced in a BackendConfig to apply protection to specific backends.
To enable a security policy, add its name to the BackendConfig. The following example configures a security policy named security-policy:
apiVersion: cloud.google.com/v1
kind: BackendConfig
metadata:
namespace: cloud-armor-how-to
name: my-backendconfig
spec:
securityPolicy:
name: “security-policy”
User-defined request/response headers
A BackendConfig can be used to define custom request headers that the load balancer appends to requests before forwarding them to the backend services.
These custom headers are only added to client requests and not to health check probes. If a backend requires a specific header for authorization and it is absent in the health check request, the health check may fail.
To configure user-defined request headers, specify them under the customRequestHeaders/customResponseHeaders property in the BackendConfig resource. Each header should be defined as a header-name:header-value string.
apiVersion: cloud.google.com/v1
kind: BackendConfig
metadata:
name: my-backendconfig
spec:
customRequestHeaders:
headers:
- “X-Client-Region:{client_region}”
- “X-Client-City:{client_city}”
- “X-Client-CityLatLong:{client_city_lat_long}”
apiVersion: cloud.google.com/v1
kind: BackendConfig
metadata:
name: my-backendconfig
spec:
customResponseHeaders:
headers:
- “Strict-Transport-Security: max-age=28800; includeSubDomains”
Automating Kubernetes Deployments with Argo CD
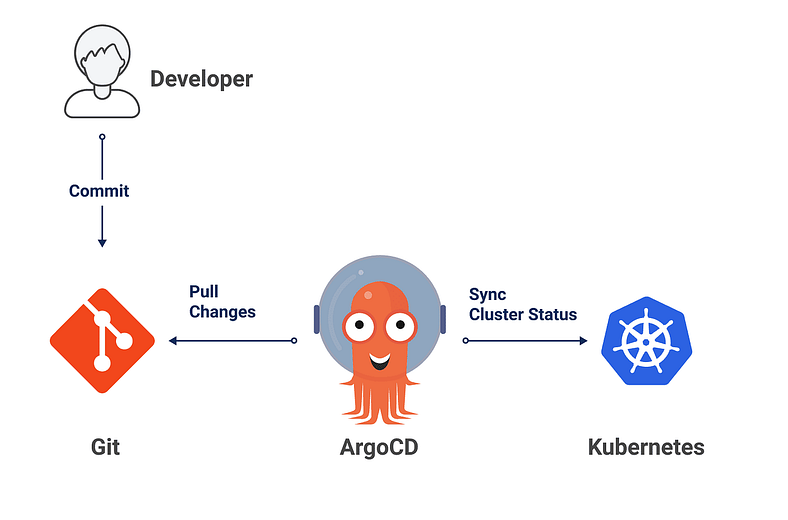
Argo CD is a declarative, GitOps-based continuous delivery tool designed for Kubernetes. It allows you to manage and automate application deployment using Git as the single source of truth. Argo CD continuously monitors your Git repository and ensures the Kubernetes environment matches the desired state described in your manifest.
Step 1: Create and Connect to a Kubernetes Cluster
Steps to Create and Connect
Create a Kubernetes Cluster
If you’re using Google Kubernetes Engine (GKE), you can create a cluster using the following command:
gcloud container clusters create <cluster name> — zone <zone of cluster>
Replace <cluster name> with your desired cluster name and <zone of cluster> with your preferred zone.
Connect to the Cluster
Once the cluster is created, configure kubectl (the Kubernetes CLI) to interact with it:
gcloud container clusters get-credentials argo-test — zone us-central1-c
Verify the connection by listing the nodes in the cluster:
kubectl get nodes
Step 2: Install Argo CD
Installing Argo CD means deploying its server, UI, and supporting components as Kubernetes resources in a namespace.
Steps to Install
Create a Namespace for Argo CD
A namespace in Kubernetes is a logical partition to organize resources:
kubectl create namespace argocd
Install Argo CD Components
Use the official installation manifest to deploy all Argo CD components:
kubectl apply -n argocd -f https://raw.githubusercontent.com/argoproj/argo-cd/stable/manifests/install.yaml
This deploys key components like the API server, repository server, application controller, and web UI.
Step 3: Expose Argo CD Publicly
By default, the argocd-server service is configured as a ClusterIP, making it accessible only within the cluster. You need to expose it for external access.
Options to Expose Argo CD
Option-1 LoadBalancer
Change the service type to LoadBalancer to get an external IP address:
kubectl patch svc argocd-server -n argocd -p ‘{“spec”: {“type”: “LoadBalancer”}}’
Ingress
For advanced routing and SSL support, create an Ingress resource. This approach is recommended if you want to add HTTPS to your setup.
Option-2 Port Forwarding
If you only need temporary access:
kubectl port-forward svc/argocd-server -n argocd 8080:80
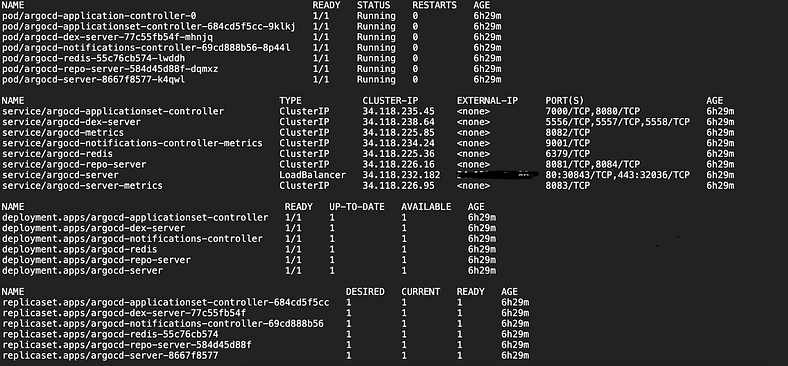
Step 4: Access the Argo CD Dashboard
Retrieve the External IP
After exposing the service as a LoadBalancer, get the external IP address:
kubectl get svc argocd-server -n argocd
Login Credentials
Username: admin
Password: Retrieve it from the secret:
kubectl get secret argocd-initial-admin-secret -n argocd -o yaml
Decode the base64 password:
echo “<base64_encoded_password>” | base64 — decode
Access the dashboard by navigating to https://<external-ip> in your browser.
Step 5: Install the Argo CD CLI
The Argo CD CLI enables you to interact with the Argo CD server programmatically for managing clusters, applications, and configurations.
Steps to Install
Download the CLI
curl -sSL -o argocd-linux-amd64 https://github.com/argoproj/argo-cd/releases/latest/download/argocd-linux-amd64
Install the CLI
sudo install -m 555 argocd-linux-amd64 /usr/local/bin/argocd
rm argocd-linux-amd64
Verify Installation
argocd version
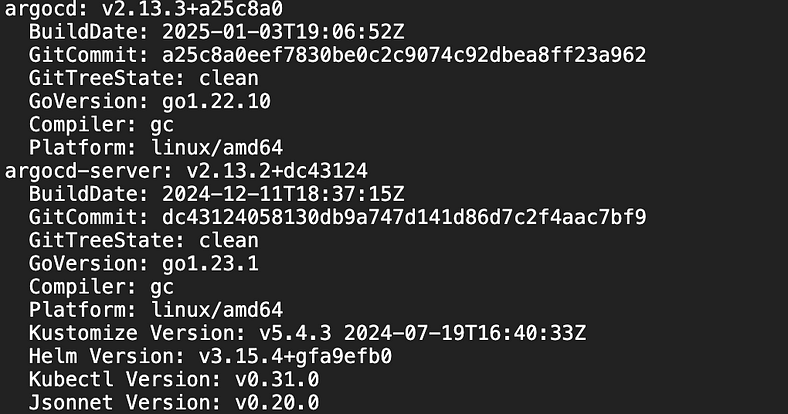
Step 6: Add a Kubernetes Cluster to Argo CD
Argo CD requires access to the Kubernetes cluster where it will deploy applications.
Steps to Add
Log in to Argo CD via CLI
argocd login <argocd-server-url>:<port> — username admin — password <password>
Get the Kubernetes Context
kubectl config get-contexts -o name
Add the Cluster
argocd cluster add <context-name>
This command creates a service account (argocd-manager) with cluster-wide permissions to deploy applications.
To Verify the added cluster via cli use below command else navigate to the ui dashboard setting -> cluster
argocd cluster list

Step 7: Add a Git Repository
The Git repository serves as the source of truth for application manifests.
Steps to Add
- Navigate to Repositories
Log in to the Argo CD dashboard, go to Settings -> Repositories, and click Connect Repo. - Enter Repository Details
- Choose a connection method (e.g., HTTPS or SSH).
- Provide the repository URL and credentials.
- Assign a project to organize repositories.
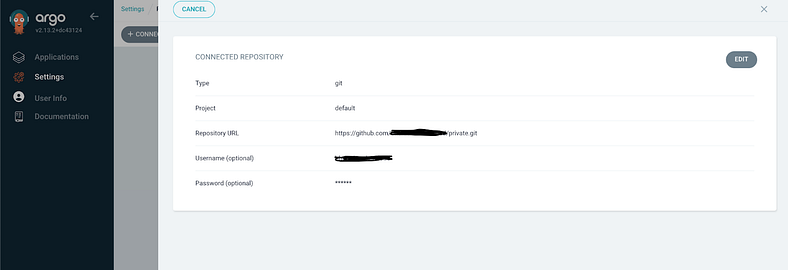
Step 8: Create an Application in Argo CD
An Argo CD application represents the Kubernetes resources defined in a Git repository.
Steps to Create
Click New App
Enter the application details:
- Application Name: e.g., hello-world
- Project: Assign the application to a project.
- Source: Select the Git repository and specify the manifest file path.
- Destination: Select the cluster and namespace for deployment.
- Enable Auto-Sync policy
Enable this option for automated synchronization between the Git repository and the Kubernetes cluster. - Create the Application
Click Create. Argo CD will deploy the application and monitor its state.
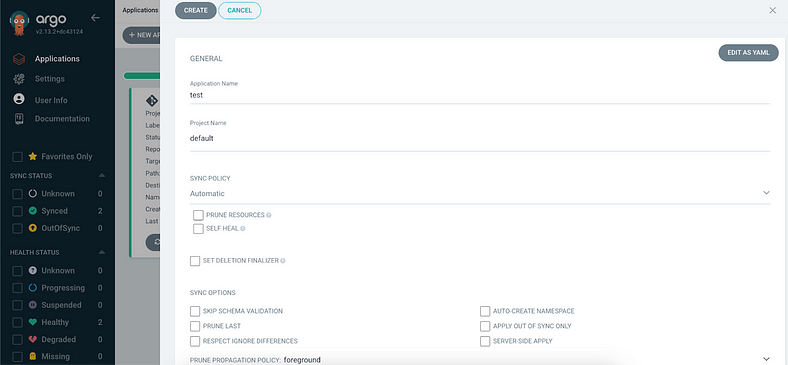
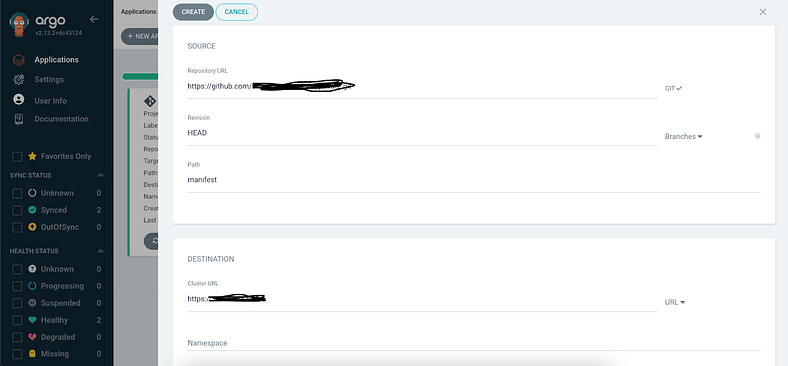
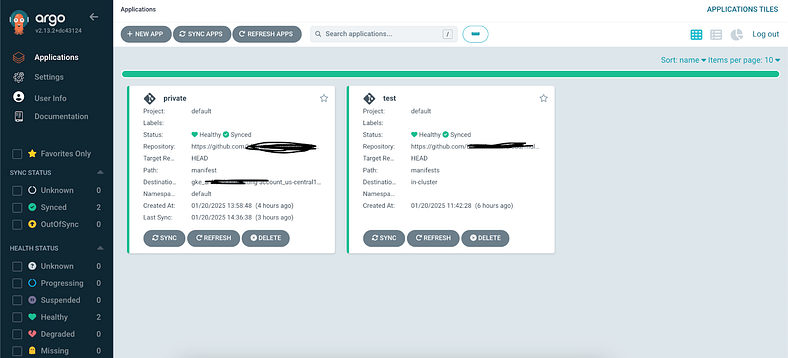
Automating S3 to GCS Migration Using Bash Scripts
Introduction
Cloud storage plays a crucial role in modern infrastructure, providing scalable and reliable storage solutions. Many businesses migrate from AWS S3 to Google Cloud Storage (GCS) to leverage cost benefits, integration with Google Cloud services, or optimize their cloud strategies. However, when dealing with hundreds of S3 buckets, manual migration is inefficient and time-consuming.
To streamline the process, I automated the migration using Bash scripts and Google Cloud’s Storage Transfer Service. In this blog, I’ll walk you through the steps of automating S3 to GCS migration efficiently.
Why Automate S3 to GCS Migration?
Handling over 200+ S3 buckets manually would involve:
- Repetitive tasks – Creating GCS buckets, setting permissions, and transferring data for each bucket.
- Human errors – Misconfiguration, incorrect bucket names, or missing files.
- Time-consuming process – Manual intervention would take days to complete.
By automating this process, we can:
Save time – Script execution takes a few minutes instead of hours/days.
Eliminate errors – Ensures all S3 buckets are correctly transferred.
Enable monitoring & scheduling – Automate recurring data transfers with Google’s Storage Transfer Service.
Prerequisites
Before running the scripts, ensure you have:
A Google Cloud Project with Billing enabled.
AWS IAM User with s3:ListBucket and s3:GetObject permissions.
Installed Google Cloud SDK (gcloud CLI) on your local machine.
Step 1: Creating Google Cloud Storage Buckets
Each S3 bucket requires a corresponding GCS bucket. The script below reads a list of bucket names from a file and creates them in GCP.
create_gcs_bucket.sh
#!/bin/bash
# Variables
PROJECT_ID="ccd-poc-project" # Replace with your GCP project ID
BUCKET_LIST_FILE="bucket_names.txt" # File containing bucket names
OUTPUT_FILE="created_buckets.txt"
REGION="us-central1" # Change if needed
# Check if the bucket list file exists
if [ ! -f "$BUCKET_LIST_FILE" ]; then
echo "Error: Bucket names file '$BUCKET_LIST_FILE' not found!"
exit 1
fi
# Read bucket names and create GCS buckets
while IFS= read -r BUCKET_NAME || [[ -n "$BUCKET_NAME" ]]; do
if [[ -z "$BUCKET_NAME" ]]; then
continue # Skip empty lines
fi
# Clean bucket name
BUCKET_NAME=$(echo "$BUCKET_NAME" | tr -d '\r' | tr -d '[:space:]')
echo "Creating bucket: $BUCKET_NAME"
gcloud storage buckets create "gs://$BUCKET_NAME" --location="$REGION" --project="$PROJECT_ID"
if [ $? -eq 0 ]; then
echo "gs://$BUCKET_NAME" >> "$OUTPUT_FILE"
echo "Bucket $BUCKET_NAME created successfully."
else
echo "Error: Failed to create bucket $BUCKET_NAME"
fi
done < "$BUCKET_LIST_FILE"
Explanation:
- Reads bucket names from bucket_names.txt.
- Cleans up any unnecessary whitespace.
- Creates GCS buckets with the specified region.
- Stores created bucket names in created_buckets.txt.
Step 2: Automating Data Transfer from S3 to GCS
After creating the required GCS buckets, the next step is to automate data transfer using the gcloud transfer jobs command.
s3_to_gcs_transfer.sh
#!/bin/bash
# Variables
AWS_ACCESS_KEY="YOUR_AWS_ACCESS_KEY"
AWS_SECRET_KEY="YOUR_AWS_SECRET_KEY"
PROJECT_ID="ccd-poc-project"
CREDS_FILE="aws-creds.json"
# Create AWS credentials JSON file
cat <<EOF > "$CREDS_FILE"
{
"awsAccessKeyId": "$AWS_ACCESS_KEY",
"awsSecretAccessKey": "$AWS_SECRET_KEY"
}
EOF
# Read bucket names and create transfer jobs
while IFS= read -r BUCKET_NAME; do
echo "Creating transfer job for S3 bucket: $BUCKET_NAME"
JOB_NAME=$(gcloud transfer jobs create s3://"$BUCKET_NAME" gs://"$BUCKET_NAME" \
--source-auth-method=AWS_SIGNATURE_V4 \
--source-creds-file="$CREDS_FILE" \
--schedule-repeats-every=1d \
--project="$PROJECT_ID" \
--format="value(name)")
if [[ -n "$JOB_NAME" ]]; then
echo "Transfer job created successfully: $JOB_NAME"
else
echo "Failed to create transfer job for $BUCKET_NAME"
fi
done < bucket_names.txt
# Remove credentials file for security
rm "$CREDS_FILE"
echo "All transfer jobs created successfully!"
Explanation:
- Generates a secure AWS credentials file.
- Reads bucket names and initiates a transfer job.
- Checks if an existing transfer is running before creating a new one.
- Deletes the credentials file after execution for security.
Step 3: Running the Migration
To execute the scripts, follow these steps:
- Save the S3 bucket names in a file named bucket_names.txt.
- Run the GCS bucket creation script:
chmod +x create_gcs_bucket.sh
./create_gcs_bucket.sh
- Run the S3-to-GCS transfer script:
chmod +x s3_to_gcs_transfer.sh
./s3_to_gcs_transfer.sh
Conclusion
By automating S3 to GCS migration, we:
Eliminated manual effort for creating 200+ buckets.
Ensured accurate and efficient data transfers.
Scheduled daily syncs for incremental updates.
This solution scales easily and can be modified to include advanced features like logging, monitoring, and notifications.
If you found this guide helpful, feel free to share your thoughts and experiences in the comments. Happy migrating!
Google Cloud - Security Alerts Automation
In this blog, we will guide you through automating alerting for critical activities and securing your projects against accidental deletion using custom scripts. Setting up log-based metrics and alerts manually can be a time-consuming task, typically taking around an hour or might be manual errors. To optimize this process and enhance efficiency, we have automated it using a combination of Shell and YAML scripts.
By implementing this solution, you can configure notification channels to receive alerts whenever changes are detected in your cloud environment, ensuring prompt action on potential issues. Our approach involves leveraging YAML files along with the Deployment Manager to create and manage Log Metrics and Alerting Policies. Once these components are successfully deployed, the deployment itself is deleted since it does not interfere with any ongoing services or resources in your cloud environment.
The following steps will provide you with a detailed, step-by-step guide to implementing this automation effectively, allowing you to maintain better security and operational efficiency.
Step-by-step guide to implementing this automation effectively
1. Clone the Repository
Prerequisites:
Connect to your Google Cloud Shell and ensure you have the necessary permissions to implement the script.
git clone https://github.com/nvrvenkat/Securitylogalerts.git
This command clones the Securitylogalerts repository from GitHub to your local system.
2. Navigate to the Repository Directory
cd Securitylogalerts/
This command changes the directory to the Securitylogalerts folder, where all project files are located.
3. Description of Metrics and Alerts
4. Replace the Email Address in logmetric_notification.sh
Update the email address in the shell script “logmetric_notification.sh” with the specific email address where alerts need to be sent.
This email address will be used to configure the notification channel.

5. Execute the Notification Channel Script
./logmetric_notification.sh
Runs the script to create a notification channel with the updated email address.
It will create a notification channel with the updated email address and generate log-based metrics as specified in the "Metrics and Alerts" section.
Note: If a notification channel already exists, execute the logmetric.sh file to generate only the log-based metrics.

6. Navigate to the Log Alert Directory and Execute Scripts
a) cd /Securitylogalerts/Logalert
./scripts.sh
The scripts.sh script triggers:
replace_notification_channel.sh: Replaces the notification channel with ACT-MS-alerts in the YAML files used for creating log metric alerts. The output is saved to output.txt.
logalert.sh: Creates alerting policies based on the updated notification channel in the YAML files.
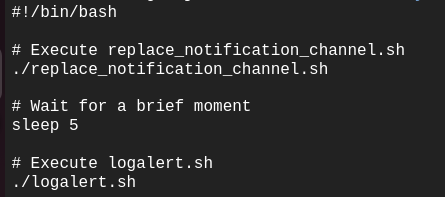
Alerting Policies Update:
- Once scripts.sh is executed, the notification channel in the YAML files will be replaced, and the alerting policies will be created.
- The alerting policies should be fully deployed within approximately 10 minutes.

The resources will be created using the Deployment Manager in the Google Cloud Console. Once the resources are created, the deployment will be deleted while retaining the resources.
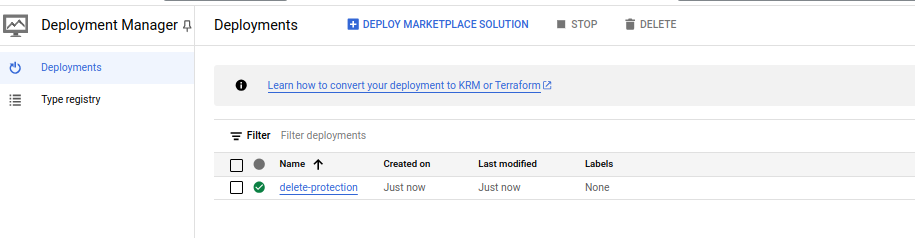
b)Add Multiple Notification Channels (optional):cd /Securitylogalerts/Logalertmultiple./scripts.sh
This command adds multiple notification channels to the alerting policies. Ensure you update the respective notification channel names in the “replace_notification_channel.sh” file before executing the script.It updates the YAML files for log alert metrics with the additional notification channels.
7. Test Alerting Policies
This script tests the alerting policies by:
- Creating and deleting resources (e.g., instances, disks, service accounts, service account keys, and firewall rules).
- Sending alerts to the configured notification channel to verify that the policies are functioning correctly.
8. Resource Creation and Deletion Activity
After executing “alerttest.sh”, resources are automatically created and deleted as per the alerting policy configurations.
Alerts are triggered and sent to the configured notification channel.
For example: Alerts for service account key creation and deletion.
Similar alerts for other resources will be triggered based on resource creation.


9.Enable Project Liens:
cd /Securitylogalerts/
./Liens.sh
Executes the “Liens.sh” script, which fetches the project ID automatically and enables liens on the project to prevent accidental deletion.
By following these steps, you'll be able to automate your cloud environment’s monitoring and security processes, ensuring that you stay ahead of any potential data and revenue losses and minimize the risk of accidental deletions.
How to Secure Your Google Cloud Buckets with IP Filtering
In today's cloud-driven world, sensitive data should be kept as secure as possible. IP filtering allows you to control who accesses your storage by enabling it on your Google Cloud buckets so that only trusted networks are allowed to access. This guide will walk you through the step-by-step process for setting up IP filtering.
What is IP Filtering?
IP filtering limits access to a bucket by allowing access only from particular IP ranges or networks. It grants access to your data while blocking traffic requests from unknown or malicious sources.
Key Use Cases for Google Cloud Bucket IP Filtering
1. Compliance Requirements
- Description: Make sure only authorized users can access the bucket to meet legal or industry rules for protecting data.
- Key: Regulatory Adherence (Following Data Protection Rules)
2. Protect Public Buckets
- Description: Enhanced security prevents unauthorized access to publicly accessible buckets by limiting traffic to trusted IPs. This protects sensitive public resources from malicious activity.
- Key: Access Control for Public Data
3. VPC Integration
- Description: Private networking limits bucket access to specific Virtual Private Cloud (VPC) networks. This ensures secure interactions within a well-defined network boundary, enhancing data protection.
- Key: Network-Specific Access
4. Controlled Testing
- Description: Access restriction during testing phases ensures that bucket access is limited to only select IPs or systems. This maintains control over the testing environment and reduces unintended data exposure.
- Key: Testing Environment Control
5. Enhanced Monitoring
- Description: Simplifies audits by restricting access to known and trusted IPs. That is, by allowing access only from trusted IPs, you reduce the number of unknown or suspicious interactions. This makes it easier to track who accessed the bucket and when, simplifying audits and improving transparency.
- Key: Simplified Audit Trails
Supported locations
Bucket IP filtering is available in the following locations:
- asia-south1
- asia-south2
- asia-southeast1
- asia-southeast2
- asia-east1
- asia-east2
- europe-west1
- europe-west2
- us-central1
- us-east1
- us-east4
- us-west1
Limitations
Bucket IP filtering has the following limitations:
- Maximum number of IP CIDR blocks: You can specify a maximum of 200 IP CIDR blocks across public and VPC networks in the IP filter rule for a bucket.
- Maximum number of VPC networks: You can specify a maximum of 25 VPC networks in the IP filter rules for a bucket.
- Dual-region support: IP filtering is not supported for dual-regional buckets.
- Blocked Google Cloud services: Enabling IP filtering on Cloud Storage buckets restricts access for some Google Cloud services, regardless of whether they use a service agent to interact with Cloud Storage.
How to Enable Bucket IP Filtering
Step 1: Install the Google Cloud CLI (Command Line Interface) in the server
SSH into your instance and install the Google Cloud CLI using the following command:
sudo snap install google-cloud-cli --classic
Authenticate with Google Cloud:
gcloud auth login

You will be prompted to grant access to your Google account.

Google Cloud SDK wants to access your Google Account

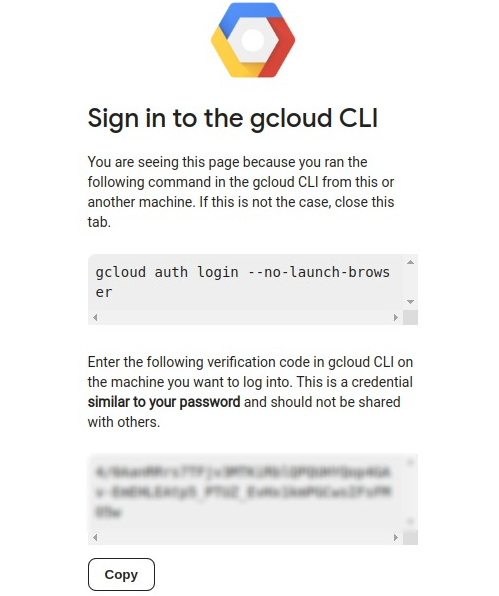


Set the desired project ID:
gcloud config set project [PROJECT_ID]
Step 2: Verify Your Bucket
- List all the buckets in your project: gcloud storage buckets list
- Locate the bucket name you want to configure.
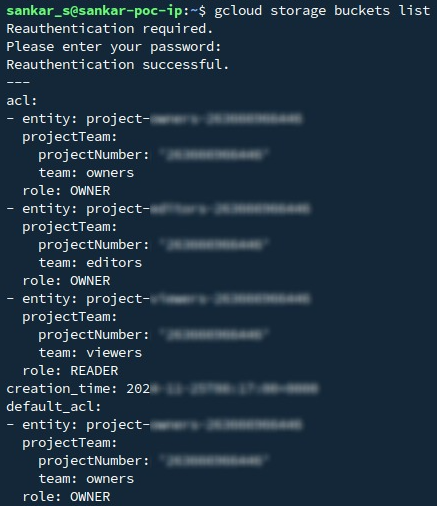
Step 3: Prepare the JSON Configuration
Create a JSON file to define your IP filtering rules:
- Open a text editor to create the file:
nano ip-filter-config.json or vim ip-filter-config.json
- Add the following configuration and save the file:
{
"mode": "Enabled",
"publicNetworkSource":
{
"allowedIpCidrRanges": ["RANGE_CIDR"]
},
"vpcNetworkSources":
[
{
"network": "projects/PROJECT_ID/global/networks/NETWORK_NAME",
"allowedIpCidrRanges": ["RANGE_CIDR"]
}
]
}
Replace the IP ranges and VPC network details with your specific requirements.
Step 4: Apply the Configuration
Run the following command to update your bucket with the IP filtering configuration:
gcloud alpha storage buckets update [BUCKET_NAME(gsutil URI)] --ip-filter-file=ip-filter-config.json

Step 5: Verify the Configuration
After applying the rules, describe the bucket to confirm the changes:
gcloud storage buckets describe [BUCKET_NAME(gsutil URI)]
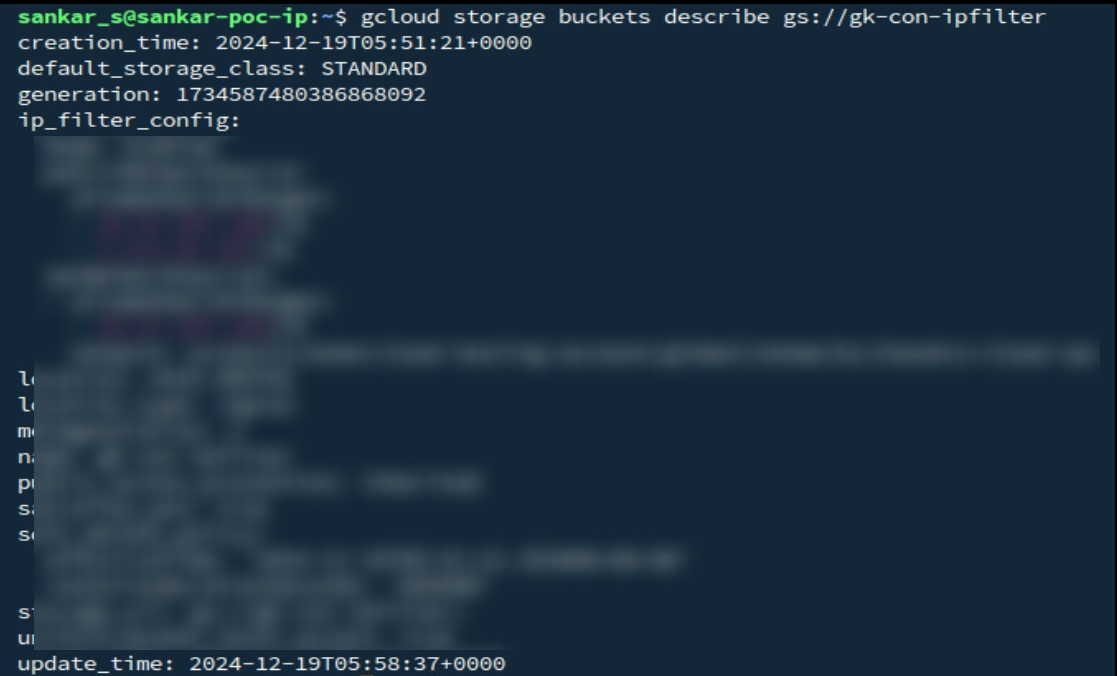
User can see the IP filter configuration in the bucket
Step 5: Test Access
- Ensure requests from allowed IPs can access the bucket.
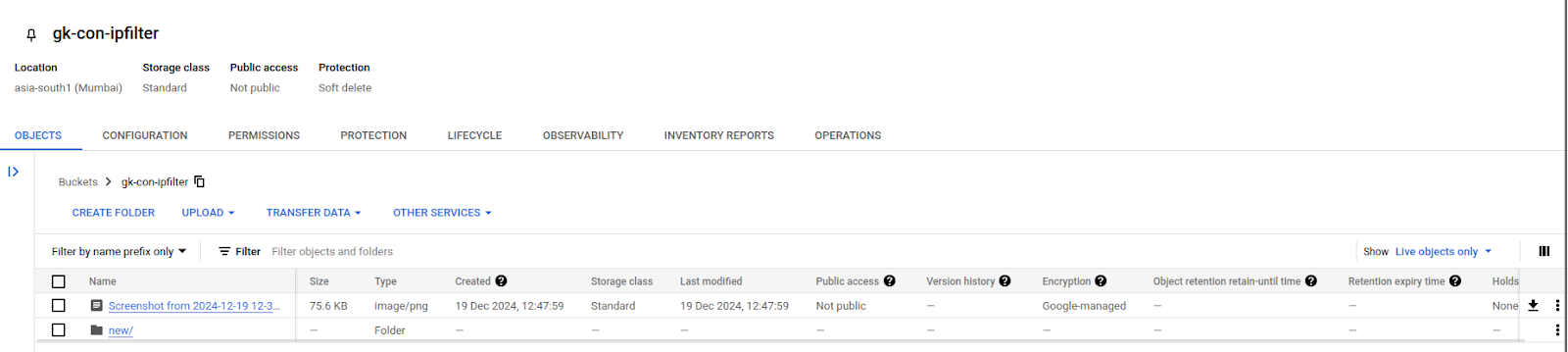
- Verify that non-whitelisted IPs are denied access.
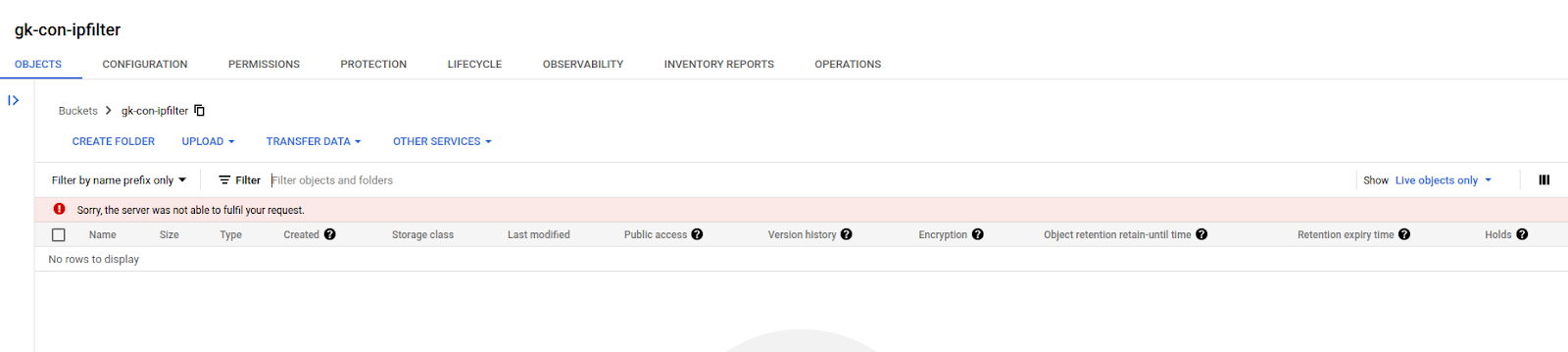
How to Disable or Remove IP Filtering Disabling IP Filtering
- If we want to disable the modify the json from “Enabled” to “Disabled” and update the bucket to apply the modify configuration.
{
"mode": "Disabled",
"publicNetworkSource":
{
"allowedIpCidrRanges": ["RANGE_CIDR"]
},
"vpcNetworkSources":
[
{
"network": "projects/PROJECT_ID/global/networks/NETWORK_NAME",
"allowedIpCidrRanges": ["RANGE_CIDR"]
}
]
}
Update the bucket with the modified configuration:
gcloud alpha storage buckets update [BUCKET_NAME(gsutil URI)] --ip-filter-file=ip-filter-config.json
Removing IP Filtering Configuration
- To remove any existing IP filtering configuration from the bucket:
gcloud alpha storage buckets update [BUCKET_NAME(gsutil URI)] --ipfiltering --clear-ip-filter
By enabling IP filtering, you can protect your Google Cloud buckets from unauthorized access and ensure compliance with organizational security policies. Whether you are securing sensitive data or limiting access during testing, these steps provide a robust framework for managing bucket security effectively.
Bypass bucket IP filtering rules:
Bypassing bucket IP filtering rules exempts users or service accounts from IP filtering restrictions for creating, deleting, or configuring buckets, while still enforcing rules for others. For more information about bucket IP filtering, see Bucket IP filtering(https://cloud.google.com/storage/docs/ip-filtering-overview).
It's crucial to have a way to regain access to your bucket if you inadvertently block your own IP address. This can happen due to the following reasons:
- Bucket lockout: When you accidentally add a rule that blocks your own IP address or the IP range of your entire network.
- Unexpected IP change: In some cases, your IP address might change unexpectedly due to network changes, and you might find yourself locked out.
To enable specific users or service accounts to bypass IP filtering restrictions on a bucket, grant them the storage.buckets.exemptFromIpFilter permission using a custom role. This permission exempts the user or service account from IP filtering rules for bucket-level operations such as creating, deleting, or configuring buckets. To do so, complete the following steps:
- Identify the user or service account that needs to bypass the IP filtering restrictions on specific buckets.
- Create a custom role.(https://cloud.google.com/iam/docs/creating-custom-roles)
- Add the storage.buckets.exemptFromIpFilter permission to the role.
- Grant the custom role to the identified user or service account at the project level.
For information about granting roles, see Grant a single role (https://cloud.google.com/iam/docs/manage-access-service-accounts#grant-single-role)
Deploying Your Project on Google Cloud: From Manual Setup to Automated CD Pipeline with Secure Git Integration
Imagine you've just put the finishing touches on your latest application. Now comes the challenging part: deploying it on Google Cloud's Compute Engine instances. The initial setup is like assembling a complex jigsaw puzzle: creating a Managed Instance Group (MIG) with auto-scaling and auto-healing, pushing your dockerized application to Artifact Registry, configuring URL maps, forwarding rules, backends, and finally, a load balancer to distribute traffic.
In the current landscape of software development, while automating code improvement remains a challenge, optimizing infrastructure management for code updates is achievable. With the involvement of iterative development methodologies, it's crucial to minimize the time and effort required for code deployment.
This blog introduces and executes an efficient Continuous Deployment (CD) pipeline leveraging Git triggers on Cloud Build within Google Cloud Platform (GCP). My approach integrates SSH key authentication, enhancing both security and automation. We'll explore how to set up a Git trigger that activates whenever changes are pushed to your repository. We'll dive into configuring Cloud Build to work with these triggers, incorporating crucial security elements like Secret Manager for handling sensitive credentials, setting up GitHub SSH keys for secure, and also setting up SSH keys to maintain secure access of your deployment on your GCP infrastructure and thus lead to seamless integration.
These steps eliminate the need for repetitive infrastructure setup with each code iteration, significantly reducing deployment overhead and enabling rapid, secure updates to your production environment.
Before jumping into the implementation, let's understand the concept of Continuous Deployment (CD) in the context of Git-based version control. CD is a DevOps practice where code changes are automatically built, tested, and deployed to production environments automatically. In the Google Cloud Platform (GCP) ecosystem, Cloud Build serves as a robust CI/CD tool that can ingest source code from diverse Version Control Systems (VCS) or cloud storage solutions, execute builds according to user-defined specifications, and generate deployment artifacts such as Docker images or Java ARchives (JARs).
Let's dive into setting up the whole CD pipeline now;
To implement GitHub triggers effectively, the initial step involves properly structuring and updating your repository with the latest codebase. It's absolutely necessary that the person configuring the Cloud Build trigger possesses the requisite permissions on the target repository. Specifically, they should have collaborator status or equivalent access rights to enable seamless integration between GitHub events and the deployment pipeline. This ensures that the CD system can respond to repository updates and initiate the deployment process. The flowchart below is an overview of the steps we will be learning and practicing today.
If you are already familiar with all the services, go ahead and complete your deployment using this diagram.
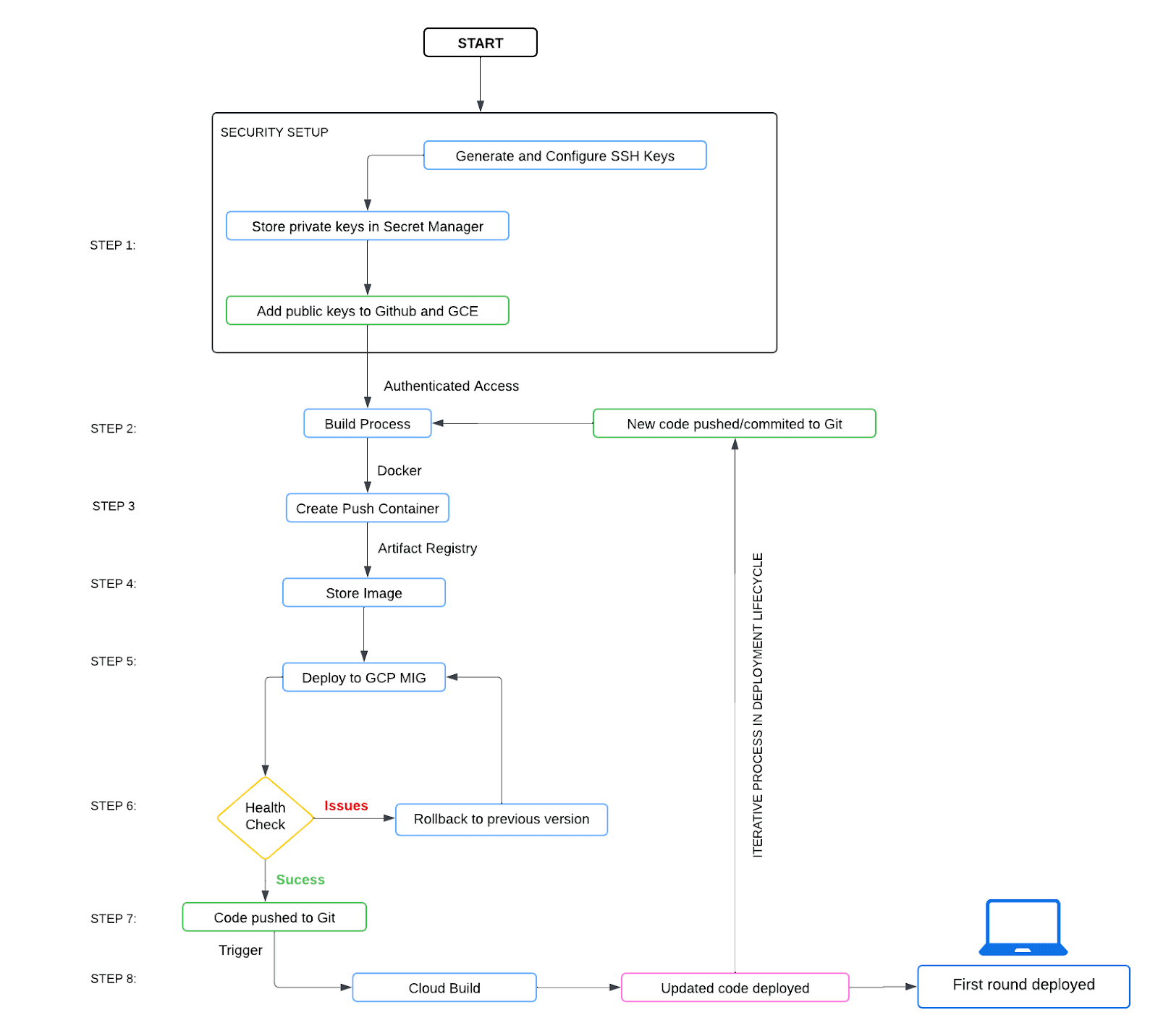
Before proceeding with the setup, ensure that the Cloud Build API and the Secret Manager API are enabled in your Google Cloud environment. These can be activated via the Google Cloud Console's API Marketplace.
Establishing GitHub SSH keys for secure repository connection
For this, open up your cloud shell on your console and wait for it to connect. Now type in the following commands:
mkdir workingdir && cd workingdir
To generate your github key run this line replace the github-email with the email id that you have used to create your repository on Github
ssh-keygen -t rsa -b 4096 -N '' -f id_github -C github-email
This generates a 4096-bit RSA key pair without a passphrase, which is crucial, as Cloud Build doesn't support passphrase-protected keys.
Secure Private Key Storage on Secret Manager
Now after the above steps you would have a private and a public Github key. The private key (id_github) must be securely stored in Secret Manager to prevent unauthorized access. To do so follow these steps:
a. Navigate to the Secret Manager in Google Cloud Console.
b. Select 'Create Secret'.
c. Assign a descriptive name to the secret.
d. For the secret value, upload the 'id_github' file from your workingdir.
e. Maintain default region settings unless specific requirements dictate otherwise.
f. Finalize by clicking 'Create secret'
Once these steps are done you can be assured that your private key is protected and isn’t accessible to everyone.
Connecting to your Github repository
Now that you have your Git keys it is necessary to add the public key on GitHub so as to connect it to your infrastructure on GCP. So log into your GitHub account move into your repository page and follow these steps:
a. Move to the Settings tab of your repository
c. In the sidebar, select 'Deploy Keys' and click 'Add deploy key'.
d. Provide a descriptive title and paste the contents of 'workingdir/id_github.pub'. This is your public key
e. Enable 'Allow write access'.
f. Confirm by clicking 'Add key'.
Once you have added the Git keys to the Secret manager and your GitHub repository Access key section you can continue and remove the local copies. This adds another level of security and makes sure nobody else is able to access your GitHub key. To do so run this on your cloud shell:
rm id_github*
Configuring Cloud Build Service Account Permissions
Now that you have the above set you need to make sure that the Service Account that you are using has access to the Secret Manager.
a. Navigate to the Cloud Build Settings page in Google Cloud Console.
b. Select the service account for your build operations.
c. Enable the 'Secret Manager Secret Assessor' role for this account.
Preparing Known Hosts for GitHub
The 'known_hosts' file is a critical component of SSH security, playing a vital role in preventing man-in-the-middle (MITM) attacks. Therefore, the final step is to set up your known hosts file.
We save the GitHub public key for SSH verification in the known_hosts file. Go ahead use this command and create a known_hosts file in the working directory
ssh-keyscan -t rsa github.com > known_hosts.github
Make sure to download the 'known_hosts.github' file to the appropriate location in the build environment, in this case your Github repository.
With the GitHub SSH keys properly configured and securely stored, the next critical step is to create your cloudbuild.yaml configuration file. This YAML file defines the series of steps Cloud Build will execute during the deployment process.
For deploying applications to Compute Engine instances via SSH, it's imperative to set up authentication keys with the appropriate access permissions. These keys will enable Cloud Build to securely push code and execute commands on your Compute Engine Managed Instance Groups (MIGs).
In the next section, we'll delve into the details of setting up these SSH keys for Compute Engine. This final piece will complete our Continuous Deployment (CD) pipeline, enabling automated deployments to Compute Engine MIGs via SSH.
Configuring SSH keys for secure access to Compute Engine instances
This step is crucial for ensuring that our Cloud Build processes can securely interact with our deployment targets. Let's walk through this process, addressing common pitfalls and best practices along the way.
1. Generating SSH Keys
Create a folder named ssh_keys on your Cloud Editor. Inside that, create a blank text file called id_rsa.txt. This is where your SSH keys will be stored: both public and private.
Let's start by generating the SSH keys. Replace the italics values in the command below and run it on your cloud shell.
ssh-keygen -t rsa -f ~/enter_path_to_id_rsa.txt -C your_username -b 2048
The addition of 2048 generates a 2048-bit RSA key pair, which offers a good balance of security and performance.
2. Enter into your instance through the shell through the following command. Now the changes and the directories of files you make will all be saved in your instance memory. Make sure that you have allotted enough memory during instance formation or MIG template formation.
gcloud compute ssh username@instance_name --zone instance_zone
3. Adding SSH Keys to Compute Engine Metadata
Once you have your key pair, you need to add the public key to your Compute Engine instance's metadata. This allows you to access the SSH on that particular instance.This can be done using the following gcloud command paste this on the:
gcloud compute instances add-metadata instance_name \
--metadata ssh-keys="username:$(location_of_public_key/id_rsa.pub)" \
--zone instance_zone \
--project project_id
Replace the name of the instance with your compute engine instance name, followed by your username, location of public key, zone in which the instance is created and finally your project id.
4. Configuring the Instance for SSH Access
Now that you have added the public key to your instance metadata, in order to access the instance you would need to add the private key in the authorized_keys file in the instance ssh folder. This private key is verified with your public key from the metadata to give access to the ssh and further processing.
On your Compute Engine instance paste the following commands to set up the authorized_keys file:
mkdir -p ~/.ssh
nano ~/.ssh/authorized_keys
The nano command opens an editor. Paste your key in this file and then save it accordingly.
Next, let's set up the correct permissions for the keys, paste these commands in the shell:
chmod 700 ~/.ssh
chmod 600 ~/.ssh/authorized_keys
These permissions are crucial for security - SSH will refuse to work if the permissions are too open.
5. Testing Your SSH Connection
Once you have executed all the above steps your SSH connection should be set. You can test your SSH connection using the verbose flag to diagnose any issues:
ssh -v -i ~/ssh_keys/id_rsa username@external_ip_of_instance
These steps lead to the completion of your CD setup and you can seamlessly integrate your code on github to your production environment.
Before you are fully ready make sure that you have docker properly installed and running in your instance. An error commonly faced while handling docker are docker authentication issues.
COMMON ISSUE
If you encounter errors like 'Unauthenticated request' when pulling Docker images, you may need to add the appropriate IAM roles. Run this command to do so:
gcloud projects add-iam-policy-binding project_id \
--member=serviceAccount:service_account_name\
--role=roles/artifactregistry.reader
Also, configure Docker to authenticate with GCR:
gcloud auth configure-docker gcr.io --quiet
With these steps you are ready with your deployment pipeline along with continuous deployment pipeline and can seamlessly integrate updates directly from your github to production environment on the Google Cloud Platform.
There might be cases where the production code that you just deployed even after multiple checks might fail and you would want to return to the previous version. This can be taken care of by the rollback method in which you can return to the previous version on your deployed code. To do this you do have an option through the console where you can choose roll back. But if you do want to continue on shell and run it though there follow this command and replace it with the correct variables.
gcloud deploy targets rollback TARGET_NAME \ --delivery-pipeline= PIPELINE_NAME \ --release= RELEASE_NAME \ --rollout-id= ROLLOUT_ID
With this do make sure that deploying bugged code on production can lead to serious downtime on your application as well as loss of data so make sure that your code is fully tested and runs smoothly with traffic before pushing it to production.
Conclusion
The art of deploying applications on Google Cloud with a secure and automated CD pipeline is more than just a technical achievement—it's a step towards streamlined, efficient development. By meticulously configuring SSH keys and leveraging Git triggers, you ensure not only the integrity of your deployment process but also the speed and reliability of your updates. This approach eliminates manual errors, reduces operational overhead, and accelerates the delivery of new features to production.
As you continue refining your cloud infrastructure, the lessons from setting up this pipeline—such as securing credentials with Secret Manager and optimizing your GitHub integration—will serve as a strong foundation. With this setup, you're not just keeping up with the fast-paced world of DevOps; you're leading the charge towards a more secure, automated future.
Supercharge Your AI Systems with Gemini 1.5: Advanced Features & Techniques
In the ever-competitive race to build faster, smarter, and more aware LLMs, every new month has a major announcement of a new family of models. And since the Gemini 1.5 family, consisting of Nano, Flash, Pro, and Ultra have been out since May 2024, many developers have already had their chance to work with them. And any of those developers can tell you one thing: Gemini 1.5 isn’t just a step ahead. It’s a great big leap forward.
Gemini is an extremely versatile and functional model, it comes equipped with a 2 million input context window. This means that prompts can be massive. And one may think that this will cause performance issues in the model’s outputs, but Gemini consistently delivers accurate, compliant, and context-aware responses, no matter how long the prompt is. And the lengths of the prompts mean that users can push large data files along with the prompt. The 2 million token input window equates to 1 hour of videos, 11 hours of audio, and 30k lines of code to be analyzed at once through Gemini 1.5 pro.
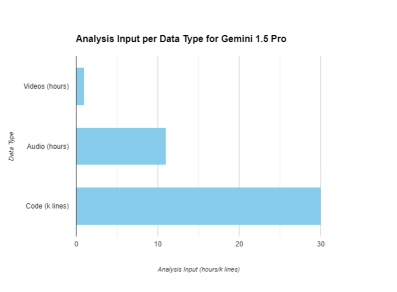
With all that being said, deciding which approach to take towards building a conversational system using Gemini 1.5 varies greatly depending on what the developer is trying to achieve.
All the methods require a general set-up procedure:
1. Google Cloud Project: A GCP project with the Vertex AI API enabled to access the Gemini model.
2. Python Environment: A Python environment with the necessary libraries (vertexai, google-cloud-aiplatform) installed.
3. Authentication: Proper authentication setup, which includes creating a service account with access to the VertexAI platform: roles such as VertexAI User. Then, a key needs to be downloaded to the application environment. This service account needs to be set as the default application credentials in the environment:
Python
import os
os.environ["GOOGLE_APPLICATION_CREDENTIALS"] = "path/to/service/account/key"
4. Model Selection: Choosing the appropriate Gemini model variant (Nano, Flash, Pro, Ultra) based on your specific needs.
Gemini models can be accessed through the Python SDK, VertexAI, or through LLM frameworks such as Langchain. However, for this piece, we will stick to using the Python SDK, which can be installed using:
Unset
pip install vertexai
pip install google-cloud-aiplatform
To access Gemini through this method, the VertexAI API must be enabled on a google cloud project. The details of this project need to be instantiated, so that Gemini can be accessed through the client SDK.
Python
vertexai.init(project="project_id", location="project_location")
From here, to access the model and begin prompting, an instance of the model needs to be imported from the GenerativeModel module in vertexai. This can be done by:
Python
from vertexai.generative_models import GenerativeModel, ChatSession, Part model_object = GenerativeModel(model_name="gemini-1.5-flash-001", system_instruction="You are a helpful assistant who
reads documents and answers questions.")
From here, we are ready to start prompting our conversational system. But, as Gemini is so versatile, there are many methods to interact with the model, depending on the use case. In this piece, I will cover the three main methods, and the ones I see as the most practical for building architectures:
Chat Session Interaction
VertexAI generative models are able to store their own history while the instance is open. This means that no external service is needed to store the conversation history. This history can be saved, exported, and further modified. Now, the history object comes as an attribute to the ChatSession object that we imported earlier. This ChatSession object can be instantiated by:
Python
chat = ChatSession(model=model_object)
Further attributes can be added, including the aforementioned chat history which allows the model to have a simulated history to continue the conversation from. This chat session object is the interface between the user and the model. When a new prompt needs to be pushed to the ChatSession, that can be accomplished by using:
Python
chat.send_message(prompt, stream=False )
This method simulates a multi-turn chat session, where context is preserved throughout the whole conversation, until the instance of the chat session is active. The history is maintained by the chat session object, which allows the user to ask more antecedent driven questions, such as “What did you mean by that?”, “How can I improve this?”, where the object isn’t clear. This chat session method is ideal for chat interface scenarios, such as chatbots, personal assistants and more.
The history created by the model can be saved later and reloaded, during the instantiation of the chat session model, like so:
Python
messages = chat.history
chat = ChatSession(model=model, history=history)
And from here, the chat session can be continued in the multiturn chat format. This method is very straightforward and eliminates the need to have external frameworks such as Langchain to manage conversations, and load them back into the model. This method maintains the full functionality of the Gemini models while minimizing the overhead required to have a full chat interface.
Single Turn Chat Method
If the functionality required doesn’t call for a multi turn chat methodology, or there isn’t a need for history to be saved, the Gemini SDK includes methods that work as a single turn chat method, similar to how image generation interfaces work. Each
call to the model acts as an independent session with no session tracking, or knowledge of past conversations. This reduces the overhead required to create the interface while still having a fully functional solution.
For this method, the model object can be used directly, like so:
Python
model = genai.GenerativeModel("gemini-1.5-flash")
response = model.generate_content("What is the capital of Karnataka?") print(response.text)
Here, a message is pushed to the model directly without any history or context added to the prompt. This method is beneficial for any use case that only requires a single turn messaging, for example grammar correction, some basic suggestions, and more.
Context Caching
Typically, LLMs aren’t used on their own, they are grounded with a source of truth. This allows them to have a knowledge bank when they answer questions, which reduces the chances that they hallucinate, or get information wrong. This is accomplished by using a RAG system. However, Gemini 1.5 makes that process unnecessary. Of course, one could just push the extracted text of a document completely into the prompt, but if a cache of documents is too large –33,000 tokens or larger– VertexAI has a method for that: Context Caching.
Context caching works by pushing a whole load of documents along with the model into a variable, and initiating a Chat Session from this cache. This eliminates the need to create a rag pipeline, as the model has the documentation to refer to on demand.
Python
contents = [
Part.from_uri(
"gs://cloud-samples-data/generative-ai/pdf/2312.11805v3.pdf",
mime_type="application/pdf",
),
Part.from_uri(
"gs://cloud-samples-data/generative-ai/pdf/2403.05530.pdf",
mime_type="application/pdf",
),
]
cached_content = caching.CachedContent.create(
model_name="gemini-1.5-pro-001",
system_instruction=system_instruction,
contents=contents,
ttl=datetime.timedelta(minutes=60),
)
The cached documents can be sourced from any GCP storage location, and are formatted into a Part object. These Part objects are essentially a data type that make up the multi turn chat formats. These objects are collated along with the prompt into a list and pushed to the Cache object. Along with the contents, a time to live parameter is also expected. This gives an expiration time for the cache, which aids in security and memory management.
Now, to use this cache, a model needs to be created from the cache variable. This can be done through:
Python
model = GenerativeModel.from_cached_content(cached_content=cached_content) response = model.generate_content("What are the papers about?")
#alternatively, a ChatSession object can be used with this model to create a multiturn chat interface.
The key advantage of the Gemini 1.5 family of models is that they have unparalleled support for multimodal prompts. The Part objects can be used to encode all types of inputs. By adjusting the mime-type parameter, the part object can represent any type of input, including audio, video, and images. Whatever the input type, the object can be appended to the input list, and the model will interpret it exactly as you need it to.
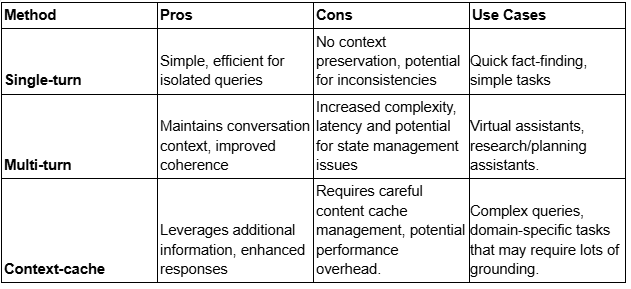
And voila! You’ve made your very own multimodal AI assistant using Gemini 1.5. Note that this is just a jumping off point. The VertexAI SDK has functionality that supports building complex Agentic systems, image generation, model tuning and more. The scope of Google’s foundational models and the surrounding support structure is ever-growing, and gives seasoned developers and newbies alike unprecedented power to build groundbreaking, superbly effective, and responsible applications.

Saasification and Cloud Migration for vitagroup: a key player in the highly-regulated German Healthcare sector

Enhancing DDoS Protection with Extended IP Block Duration Using AWS WAF Rate-Based Rules
.png)
Smart Risk Assessment: Bitech’s AI-Driven Solution for Property Insurance

Streamlining CI/CD: A Seamless Journey from Bitbucket to Elastic Beanstalk with AWS CodePipeline
.png)
Setting Up Google Cloud Account and Migrating Critical Applications for Rakuten India

Migration to Cloud and Setting Up of Analytics along With Managed Services
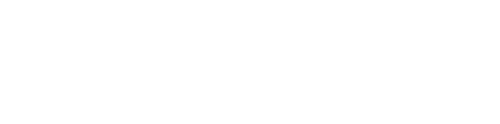
gocomo Migrates Social Data Platform to AWS for Performance and Scalability with Ankercloud
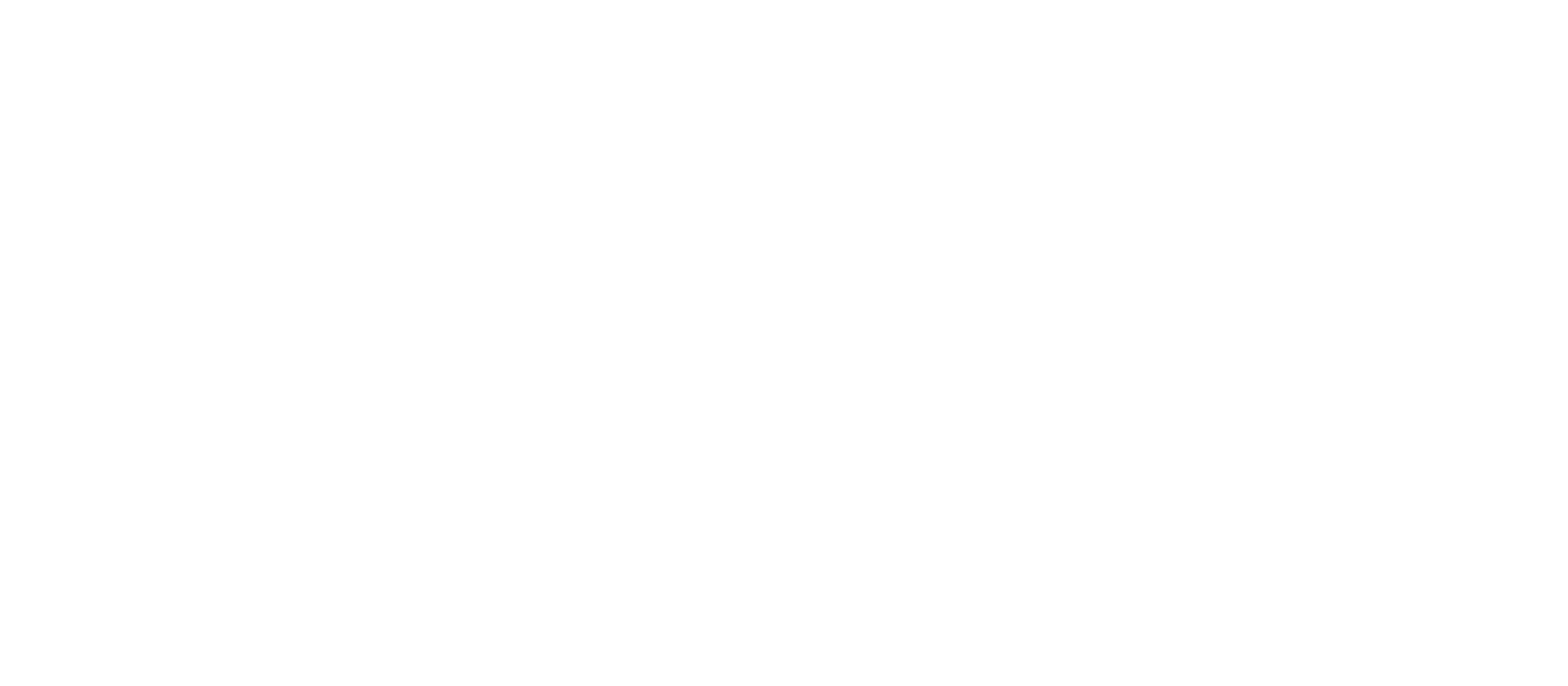
Benchmarking AWS performance to run environmental simulations over Belgium
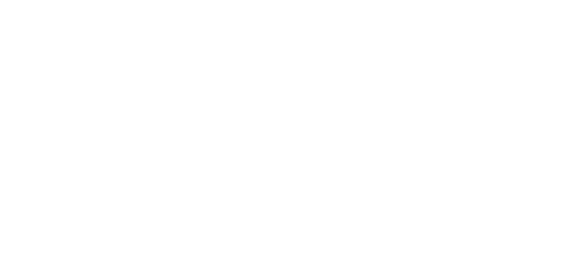
Model development for Image Object Classification and OCR analysis for mining industry

Achieving Cost Optimization, Security, and Compliance: Ankercloud's AWS CloudOps Solutions for Federmeister
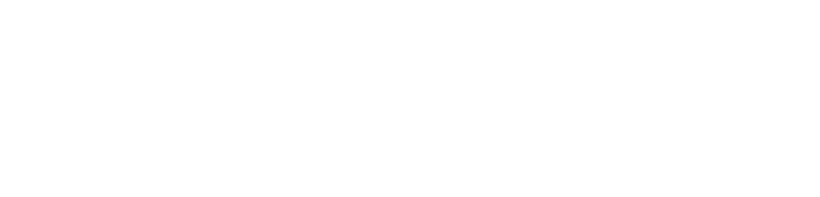
Replication of On-premise Infrastructure into AWS Cloud on Docker Swarm platform
Please Type Other Keywords
The Ankercloud Team loves to listen
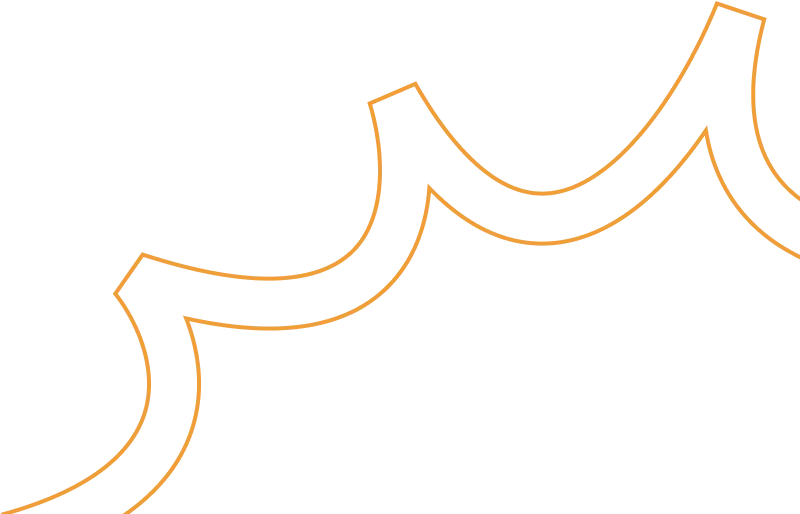
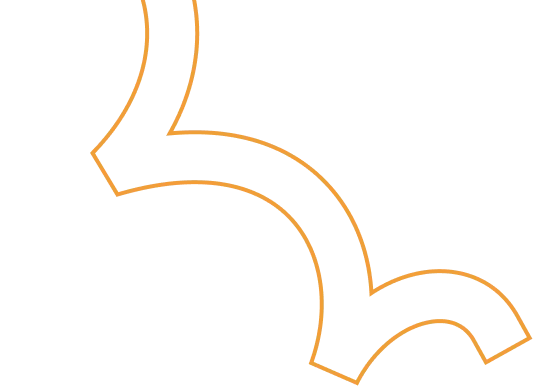