Resources
The latest industry news, interviews, technologies and resources.
How to build a Software-as-a-Service (SaaS) product on AWS
More and more companies operating in the IT sector are born with, have switched to, or are evaluating the Software-as-a-Service (SaaS) business model as an effective way to deliver their services to customers. SaaS in the cloud is the perfect solution to leverage all the available modern tools and automated processes, but how much do you know about the optimal way to build these products on AWS?
The problem
Let’s say that your company is interested in managing a SaaS product on AWS, but you are unsure how you should approach the problem or how to start implementing a new feature that needs to be integrated with the offer. Whether you are:
- Thinking about adopting a SaaS model
- Planning to onboard a lot of new customers
- Already using SaaS, on AWS or on another platform
- Working on new license-based solutions
- Looking to modernize your whole setup or a specific part of it
- Interested in improving your DevOps pipeline
… we at Ankercloud think you could strongly benefit from the AWS SaaS Discovery Program.
The solution
Being a SaaS-certified partner and benefitting from tight cooperation with AWS, Ankercloud embarks you on a discovery journey with the aim of giving you full guidance for SaaS-related innovations, customized to your needs. That’s what the SaaS Discovery Program is all about: a period of time ranging from 2–4 weeks to be spent together, starting with technical deep dive workshops to align on your specific starting point and requirements, all the way into AWS architecture design, modernization discussions, TCO computation, best practices explanation, and much more — always suited to your business case.
But the good part does not end here: depending on your growth potential, we are able to provide the SaaS Discovery Program free of charge for you (i.e. 100% discount/funding).
High Potential use cases
The focus of the SaaS Discovery Program is always to accommodate your needs and concentrate on improving your weak points. Depending on your inputs, examples of common use cases can be:
- SaaS Design Decomposition
- Authentication and Access Management
- CI/CD Pipelines
- Database Multi-tenancy and Tenants isolation
- Security and Reliability
- SaaS DevOps
- Agility and Operations
But this list is non-exhaustive, and we at Ankercloud are always open to learning about your specific obstacles and understanding how we can support you. And here is our challenge for you: bring us your most critical SaaS-related issue, we will be happy to discuss it and bring all our deep technical knowledge to develop a solution together.
What about the outcome?
This program is intended to provide flexibility and visibility during the whole planning and discovery process. Therefore, once the program is completed, there is no obligation to further continue with the implementation of the developed solution on AWS: no commitment of any kind is in fact implied, as the name discovery suggests.
Several documents and deliverables will anyway help you in the decision-making process, giving full visibility to the planned solution. At the end of the program, Ankercloud will in fact provide you with a detailed technical report with an architecture diagram, a complete analysis of the AWS costs within an 18 months time horizon, and a full proposal to continue working together with the implementation, to give us the possibility of providing further hands-on support if needed.
Sounds interesting? Are you ready to start exploring new SaaS solutions and best practices?
Don’t hesitate to contact us at: cloudengagement@ankercloud.com
Let us guide you through the steps and check your eligibility for the SaaS Discovery Program.
Introducing ACE — our Accelerated Cloud Exploration program!
Do you have too much data to handle and analyze?
Are your IT budgets maxed out and you are unsure if Cloud is a good alternative?
Are you uncertain if Cloud aligns with your security requirements and can align with business processes?
When it comes to migrating to the cloud there are many different scenarios and challenges our customers need to assess and tackle. One of the above questions can be the trigger moment to consider migrating to or modernizing within the cloud. But what does migration imply?
When we talk about migration it could be the traditional case of a full IT migration from on-prem or one cloud provider to the other, but it can also mean bringing a large workload — like a whole Machine Learning application — into an existing infrastructure on the cloud. We also talk about a migration case when a customer is planning to add a new component to existing infrastructure or is modernizing and reshaping their cloud infrastructure.
Since there are so many possible reasons to consider choosing Cloud and every requirement and use case is unique, we have developed a new program — the Accelerated Cloud Exploration (ACE) — to help our customers assess their status quo and get full visibility on relevant stakeholders, timelines, a detailed analysis of Cost of Ownership (TCO) along with a Testbed/Sandbox when considering migrating to the cloud.
What is it?
ACE contains the components of the AWS MAP Assess phase and combines them with the substantial migration expertise and experience of Ankercloud as well as the speed and agility that we can provide through the strength of our global team.
How does it work?
The program runs in a 4–6-week time frame in which we conduct several workshops, deep dive sessions and prepare testbeds/Sandboxes together with our customers, and create a detailed report which provides you with all aspects of cloud adoption for your needs.
What is Included?
· Migration Readiness Assessment — The first workshop focuses on examining the scope and targets of a potential migration as well as shedding light on the current platform setup, governance, and security requirements by analyzing our customers’ readiness/adoption factors.
· Discovery Workshop — Once we have the business, product, and organizational alignment, we move our focus to the current technology inventory like the existing application stack and databases to then start mapping the right services and infrastructure on AWS.
· Migration Patterns and Architectures — After the Discovery Workshops, we built an exact AWS architecture that would suit your needs. We create the exact architectural diagrams, configurations and systems that enable them to adopt new cloud services or replace existing infrastructure with AWS.
· Total Cost of Ownership (TCO) Analysis –Using this architecture and understanding of your utilization, we develop an investment plan and ROI analysis for the next 36 months by accounting for post-migration AWS costs, saving costs from alternative options, and providing the correct infrastructure sizing and configurations.
· Proof of Concept (POC) — While the previous phases of this program focus on helping you get complete visibility of all facets of cloud adoption, we go one step further to help you get a direct hands-on taste of it. Within ACE, we also include a PoC to provide our customers with a sandbox environment or application on AWS to experience the advantages of a migration firsthand and get their developers a “look and feel” of their post-migration infrastructure.
· Carbon Emission Calculation — In every MAP Assess project we make use of the AWS Carbon Footprint tool which allows us to include detailed calculations and comparisons of on-prem vs. AWS CO2 emissions into the report and highlight CO2 savings for the customer.
How Much Does ACE Cost?
Depending on your current and future IT Infrastructure plans, we can provide ACE program free of charge (i.e. 100% discount/ funding).
Furthermore, after this program there is further incentivization in working with us — any follow-up activities that you would like to work on with us, for example — database and server migration, application migration, and creation of various IT environments are discounted by 50%.
And there’s more — If you do choose to migrate your workloads to AWS after the ACE program, you get 25% off on your AWS bills towards any new migrated workload for the first 36 months.
Sounds Interesting?
Our ACE Program, in collaboration with AWS, is the perfect way to start exploring the cloud as the next step in your IT or Product expansion and scaling plans. And you can now make that decision with an experienced external partner with potentially zero costs. If that sounds like an exciting proposition reach out to us at cloudengagement@ankercloud.com
Unraveling the Magic: what’s the power behind AI/ML and how to release it with AWS SageMaker
What is AI?
AI (Artificial Intelligence) refers to the field of computer science that focuses on creating intelligent machines capable of mimicking and performing tasks that typically require human intelligence. AI encompasses various subfields, such as machine learning, natural language processing, computer vision, and robotics.
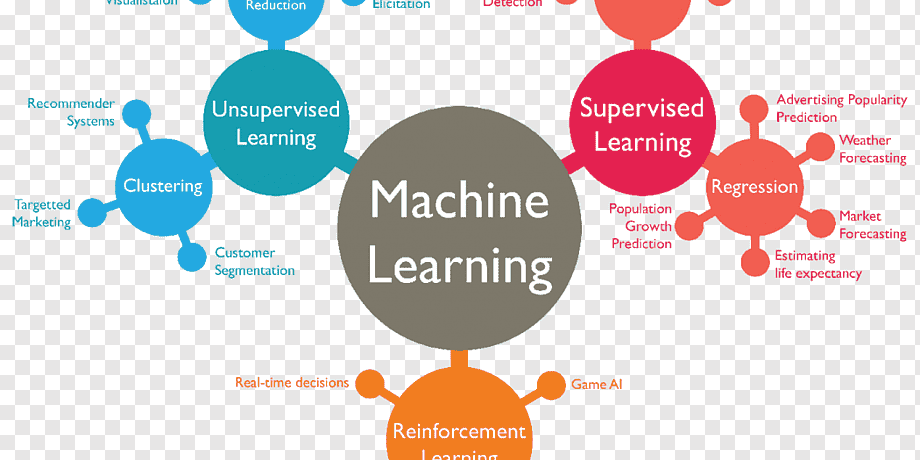
What is ML?
ML (Machine Learning) is a subset of AI that involves the development of algorithms and models that enable computers to learn from and make predictions or decisions based on data. Instead of being explicitly programmed, machine learning systems are trained on data and use statistical techniques to identify patterns and make informed decisions or predictions.
How AI/ML Works Using AWS Services?
1. Data Collection and Storage:
The foundation of AI/ML lies in data. AWS offers various services like Amazon S3, Amazon RDS, or Amazon DynamoDB for collecting, storing, and managing vast amounts of data. These services ensure secure, scalable, and reliable storage of the data required for AI/ML projects.
2. Data Preprocessing and Cleaning:
Data preprocessing is a crucial step to ensure high-quality input for ML models. AWS services like AWS Glue and AWS Data Pipeline assist in transforming, cleaning, and normalizing the data. These services automate the data preparation process, making it more efficient and accurate.
3. Training ML Models:
The training phase involves building ML models by providing data and desired outcomes. AWS SageMaker is a powerful service that simplifies the ML model training process. It offers a collaborative development environment, built-in Jupyter notebooks, and support for popular ML frameworks like TensorFlow and PyTorch.
4. ML Model Deployment:
Once the ML model is trained, it needs to be deployed to make predictions or provide insights. AWS SageMaker makes model deployment easy with its managed hosting service. Users can deploy their models as endpoints, which automatically scale based on demand.
5. Model Monitoring and Optimization:
After deployment, monitoring the ML model’s performance is crucial to ensure its accuracy and effectiveness. AWS CloudWatch integrates with SageMaker to provide real-time monitoring and alerts for model performance, resource utilization, and potential issues. This allows businesses to optimize models, detect anomalies, and address any drift in model behavior.
6. Automated ML with AWS AutoML:
AWS offers AutoML services like Amazon Comprehend and Amazon Forecast, which simplify the process of ML model development and deployment. These services use pre-built ML models and automatically train and optimize them based on the specific use case. AutoML makes AI/ML accessible to a wider audience, even those without deep ML expertise.
7. Data Visualization and Insights:
AWS QuickSight is a powerful business intelligence service that enables businesses to derive insights from their data and visualize them through interactive dashboards. QuickSight integrates seamlessly with various AWS data sources and ML models, allowing users to explore patterns, trends, and correlations in the data.
8. Advanced AI Services:
AWS offers a range of advanced AI services that leverage deep learning and natural language processing (NLP). Services like Amazon Rekognition provide image and video analysis, while Amazon Comprehend offers NLP capabilities for sentiment analysis, language detection, and entity recognition. These services enable businesses to extract valuable information from unstructured data and enhance their applications.
How Sagemaker Revolutionized the AI/ML World?
In the rapidly evolving field of Artificial Intelligence (AI) and Machine Learning (ML), staying ahead of the curve requires powerful tools and platforms that streamline the development and deployment of models. One such game-changer is Amazon SageMaker. Developed by Amazon Web Services (AWS), SageMaker has emerged as a revolutionary cloud-based platform, empowering data scientists and developers to build, train, and deploy ML models with unparalleled ease and efficiency.
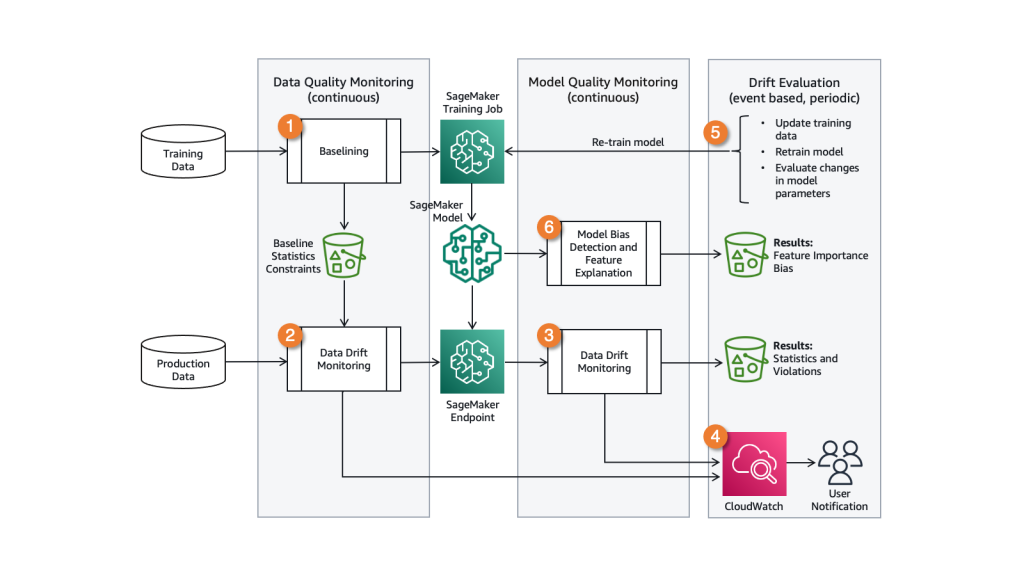
Here are some of the key impacts of SageMaker:
Simplified ML Workflow: SageMaker simplifies the end-to-end ML workflow by providing a unified environment for data preprocessing, model training, deployment, and inference. It offers a range of built-in algorithms and frameworks, as well as tools for data labeling and model tuning, making it easier for developers and data scientists to build and deploy ML models.
Scalability and Efficiency: With SageMaker, users can easily scale their ML workloads to handle large datasets and complex models. It automatically provisions the required compute resources and optimizes the training process, reducing the time and effort required to train models at scale.
Cost Savings: SageMaker offers cost-effective pricing models, such as pay-as-you-go and spot instances, allowing users to reduce ML infrastructure costs. It also provides features like automatic model scaling and resource optimization, further optimizing costs by using resources efficiently.
Built-in Tools and Frameworks: SageMaker includes a variety of pre-built ML algorithms, frameworks (such as TensorFlow and PyTorch), and optimization tools. These tools simplify the development process and enable users to quickly prototype and experiment with different models and techniques.
Easy Deployment and Management: SageMaker streamlines the deployment process by providing pre-built containers and managed hosting. It enables seamless integration with other AWS services, allowing users to easily deploy ML models as web services or integrate them into existing applications.
Collaboration and Reproducibility: SageMaker facilitates collaboration among teams by providing shared notebooks and version control integration. It allows multiple data scientists to work on the same project simultaneously, improving productivity and reproducibility.
Robust Infrastructure: SageMaker leverages the scalable infrastructure of AWS, ensuring high availability and reliability for ML workloads. It provides built-in monitoring and logging capabilities, enabling users to track model performance and troubleshoot issues effectively.
Advantages of AI/ML in Business:
1. Enhanced Efficiency and Automation:
AI/ML technologies have the potential to automate repetitive and mundane tasks, allowing businesses to optimize their operational efficiency. By automating processes like data analysis, customer support, and inventory management, companies can reduce costs, save time, and allocate resources more effectively.
2. Improved Decision Making:
AI/ML algorithms can process vast amounts of data and extract valuable insights. By analyzing patterns and trends, businesses can make data-driven decisions with greater accuracy and speed. This enables organizations to identify opportunities, predict market trends, and optimize their strategies for better outcomes.
3. Personalized Customer Experience:
AI-powered tools enable businesses to offer personalized experiences to their customers. By analyzing customer behavior, preferences, and purchasing history, companies can deliver targeted marketing campaigns, personalized product recommendations, and tailored customer support, resulting in increased customer satisfaction and loyalty.
4. Advanced Data Analytics:
AI/ML technologies can uncover hidden patterns and correlations within large datasets, providing valuable insights for businesses. These insights help identify customer preferences, market trends, and emerging risks, allowing organizations to gain a competitive edge. Data analytics powered by AI can also streamline decision-making processes and identify areas for improvement.
Disadvantages and Challenges of AI/ML in Business:
1. Lack of Human Touch:
One of the key challenges of AI/ML is the potential loss of the human touch in customer interactions. While AI-powered chatbots and virtual assistants can handle routine inquiries, they may struggle to replicate the empathy and understanding that comes from human interaction.
2. Data Privacy and Security Concerns:
AI/ML relies heavily on data, often involving sensitive customer information. This poses potential risks related to data breaches, privacy infringements, and misuse of personal data. Businesses must prioritize robust data protection measures and adhere to ethical practices to ensure the security and privacy of their customers’ information.
3. Initial Investment and Technical Challenges:
The initial costs can be high, especially for small and medium-sized enterprises (SMEs). Additionally, integrating AI/ML into existing systems and processes may pose technical challenges and require reengineering, causing disruption during the transition phase.
Why is AI Needed in Today’s World?
1. Increasing Complexity of Data:
As businesses generate and collect vast amounts of data, traditional methods of analysis and decision making are becoming inadequate. AI/ML technologies possess the ability to process and analyze complex datasets efficiently, providing valuable insights that humans may miss. This helps organizations make more informed decisions in real-time.
2. Competitive Advantage:
In today’s highly competitive marketplace, businesses need to stay ahead by offering innovative products, personalized services, and exceptional customer experiences. AI/ML enables companies to gain a competitive advantage by driving operational efficiency, improving customer satisfaction, and facilitating informed decision making.
3. Addressing Scalability and Resource Constraints:
AI/ML technologies can help businesses scale their operations by automating tasks, optimizing workflows, and reducing the reliance on human resources.
Conclusion:
AWS services have revolutionized the way AI/ML works, making it more accessible, scalable, and efficient for businesses. From data collection and preprocessing to model training, deployment, monitoring, and optimization, AWS provides a comprehensive ecosystem for AI/ML workflows. By leveraging these services, businesses can unlock the full potential of AI/ML, drive innovation, and gain a competitive advantage in the digital era.
Unleash high-performance data streaming using Amazon Kinesis
Every day we generate 2.5 quintillion bytes of data. However, only 1% of the data is processed into meaningful information due to lack of compute power. Among which most of it is generated as streaming data from a large number of sources such as social media feeds, IT logs, IoT telemetry data, online gaming, financial transactions, etc.
Streaming data is continuously generated from various sources. The stream processing technology is utilized to process streaming data in real-time. However, It is expensive to set up a large compute capacity and storage facility to process and store the streaming data.
To address this problem Amazon Web Services offers Amazon Kinesis a versatile service as the solution for challenges faced in handling and processing streaming data.
What is Amazon Kinesis?
Amazon Kinesis is a fully managed, scalable service that can ingest, buffer, and process streaming data in real-time.
Amazon Kinesis Services include Kinesis Data Stream, Kinesis Firehose, Kinesis Video Stream, and Kinesis Data Analytics.
1. Amazon Kinesis Data Streams
Kinesis Data Streams is a real-time streaming service that captures gigabytes of data from hundreds and thousands of data sources. Data streams are divided into one or more shards, each of which provides a fixed unit of capacity. Shard is the unit of base throughput for Amazon Kinesis Data Streams.
To create a stream, the number of shards has to be predefined. Each shard can support up to 5 transactions per second for reads, up to a maximum total data read rate of 2 MB per second. And up to 1,000 records per second for writes, up to a maximum total data write rate of 1 MB per second.
The total capacity of the stream is the sum of the capacities of its shards. An increase in the number of shards results in high processing speed and capacity of the data stream. Kinesis Data Streams cannot scale up in real-time if the data throughput is higher than shard capacity.
Kinesis Data Streams High-Level Architecture:

Benefits:
- Real-time performance: Streaming data is available to multiple real-time analytics applications, Amazon S3, and AWS Lambda within 70 milliseconds of the data being collected.
- Data retention: Data can be retained for seven days, the default is 24 hours.
- Secure: Data can be secured at-rest by using server-side encryption, AWS KMS master keys, and by privately accessing your data via Amazon Virtual Private Cloud (VPC).
- Low cost: Kinesis Data Streams has no upfront cost, and you only pay for the resources you use.
2. Amazon Data Firehose
Amazon Data Firehose is a near real-time service that takes care of almost everything that’s needed in capturing, transforming, and storing the data. It is a fully managed service that automatically scales to allow an increase or decrease in data throughput with no administration.
Firehose has a minimum latency of 60 seconds. It can transform and compress the data before loading it. The supported compressed formats are GZIP, ZIP, and Snappy when the target is S3.
Amazon Data Firehose provides effortless ingestion of data to data lakes such as S3, Amazon ElasticSearch, Amazon Redshift, and Splunk.
It allows the conversion of data formats before ingesting into any of the storage services, usually from JSON to Parquet or ORC and that’s only for storage in S3. It doesn’t directly convert CSV to Parquet or other formats, an AWS Lambda function can be triggered to convert CSV to JSON.
Amazon Data Firehose High-Level architecture:

Benefits:
- Easy to use: With just a few clicks Amazon Data Firehose can be set up from AWS management console.
- Pay for only what you use: It costs only for the amount of data that is streamed. It is much cheaper compared to Kinesis Data Stream showing about 60+% saving.
- No ongoing administration: It automatically provisions, scales compute capacity, memory, and network resources required to load the streaming data.
- Serverless data transformation: Process, transform, and compress prior to downloading it to data stores.
3. Amazon Kinesis Data Analytics
Amazon Kinesis Data Analytics is a service for ETL using SQL queries on the streaming data. It analyzes and provides insights in real-time. Kinesis Data Analytics implements Amazon’s state of the art Random Cut Forest for anomaly detection. Another machine learning tool Hotspots locates and returns information about relatively dense regions in the data.
The architecture of Amazon Kinesis Data Analytics:

Benefits:
- Real-time analytics and ML: Powerful real-time analytics for ETL, and built-in real-time anomaly detection using ML.
- No servers to manage: It runs your streaming applications without requiring you to provision or manage any infrastructure.
- Pay only for what you use: pay only for the processing resources that your streaming applications use.
4. Amazon Kinesis video streams
Amazon Kinesis video streaming is a high performance and efficient streaming service for videos with very low latency rates. Videos can be streamed effortlessly in a secure manner from sources like security cameras and be stored in data stores for further analysis.
AWS AI services such as Amazon Rekognition can be utilized to get real-time insights and predictions as well as create meta data of the video streams.
The architecture of Kinesis video streams:

Benefits:
- Amazon Kinesis video stream is serverless and therefore saves the customer from administrative and service management overhead costs.
- Capacity to stream from millions of devices as well as build real-time applications.
Use Case of Amazon Kinesis
The architecture below depicts a use case that implements the Amazon Kinesis Services for real-time video surveillance.

The surveillance footage from the security camera is ingested by Amazon Kinesis Video Streams.
Amazon Rekognition (a service for object and face detection) is implemented to identify faces in real-time from the video footage. Amazon Rekognition can identify known and unknown faces by comparing the faces in video streams against the faces in the S3 bucket. It can also provide the identifying features without the actual footage of faces only in the S3, where privacy is quintessential.
Amazon Kinesis Firehose streams the analyzed data from Amazon Rekognition to S3 bucket. A Lambda Function is invoked to update the face collection database every time a face is processed and stored in the S3 bucket. Simultaneously, The data analyzed by the Amazon Rekognition is written to Kinesis Data Streams which can trigger a Lambda function and invoke SNS to create a notification to the security system. This allows for immediate action in case of any security breach.
This use case demonstrates that integration of Kinesis services allows real-time analysis, monitoring, and notification which is not feasible with batch processing methods.
Conclusion
Amazon Kinesis handles streaming data, performs ETL, and real-time ML with minimal DevOps support. Amazon Kinesis is very flexible and highly reliable which makes it a very good candidate for processing as well as building applications from real-time data.
The transition from batch processing to stream processing is of great value to businesses that rely on time-sensitive data. Amazon Kinesis services empower businesses that rely on real-time applications essential for their business decisions. This saves them from spending time and energy on deploying and managing infrastructure.
Would you like to know more about AWS Kinesis or need any assistance in building a real-time application from streaming data for your business? Contact us at info@ankercloud.com.
Ankercloud Achieves the AWS Service Delivery Designation for Amazon OpenSearch Service
We at Ankercloud are pleased to announce today that we have achieved the Amazon Web Service (AWS) Service Delivery designation for Amazon OpenSearch Service. This achievement recognizes that Ankercloud provides deep technical knowledge, experience, and proven success in delivering Amazon OpenSearch Service to customers.
Achieving the Amazon OpenSearch Service Delivery designation differentiates Ankercloud as an AWS Partner Network (APN) member, helping customers to perform interactive log analytics, real-time application monitoring, a website search, and more. To receive this designation, APN Partners must possess deep AWS experience and deliver solutions seamlessly on AWS.
“Ankercloud is proud to receive the designation for Amazon OpenSearch Service Delivery,” said Santhosh Jayaprakash, Founder & CEO. “Our team is dedicated to helping companies achieve their technology goals by leveraging the agility, breadth of services, and pace of innovation that AWS provides. This new designation is proof of the success, efforts, and hard work that every single individual at Ankercloud puts into their work on a daily basis.”
AWS enables scalable, flexible, and cost-effective solutions from startups to global enterprises. To support these solutions’ seamless integration and deployment, AWS established the AWS Service Delivery Program to help customers identify APN Consulting Partners with deep experience in delivering specific AWS services.
Amazon OpenSearch Service is a managed service that makes it easy to deploy, operate, and scale OpenSearch clusters in the AWS Cloud. At Ankercloud, we help AWS clients to foster a simple way of utilizing the search for all their operational analytics workloads.
As an AWS OpenSearch Delivery Partner, we distinguish ourselves from other competitors with solid experience and profound knowledge of AWS services. We have achieved a specialized approval process vetted by AWS experts to ensure we are following best practices with Amazon OpenSearch.
Ankercloud has a proven track record in delivering projects and utilizing AWS services like Amazon OpenSearch to help our clients across the globe. Do you have workloads that could benefit from Amazon the OpenSearch Service? Please reach out to us today and we’d be happy to explore the opportunities with you!
Ankercloud is Amazon OpenSearch Launch Partner 🚀
Amazon Web Services (AWS) has just announced the launch of the Amazon OpenSearch Service Delivery specialization to help customers find validated AWS Partners with deep technical knowledge, experience, and proven success delivering Amazon OpenSearch Service.
We are super proud to announce that Ankercloud is one of the first AWS partners globally to achieve this AWS Service Delivery designation for Amazon OpenSearch Service. This achievement recognizes that our team has a proven track record in helping our customers with use cases like the performance of interactive log analytics, real-time application monitoring, website search, and more.
➡️ Do you have workloads that could benefit from Amazon the OpenSearch Service? Please reach out to us today and we’d be happy to explore the opportunities with you!
Want to know more about our new AWS badge? Read the latest blog post!
Getting started with an AWS data lake
What is Data Lake?
A data lake is a sizable, central repository that enables businesses to store and handle enormous volumes of unstructured, raw data in a variety of formats. Many sources, such as transnational systems, social media, sensors, and more, can contribute data to a data lake.
What is AWS Data Lake?
An organization can store and handle enormous amounts of raw, unstructured data in numerous formats in a data lake, a sizable, centralised repository. A data lake may contain data from many different sources, such as transactional systems, social media, sensors, and more.
Need of Data lake :-
You should construct an AWS Data lake immediately if you are experiencing any of the difficulties listed below.
1. Companies without a single source of data and too many data storage. having trouble obtaining info from several sources.
2. The cost of storing data is out of control and data volume is growing daily.
3. The way that data is organized varies greatly. Businesses, for instance, have data from logs, IoT devices, user audits, and image galleries.
4. Big data analytics using slow data.
This would make it obvious to you whether your company needs Amazon Data Lake or not.
Services Under in AWS DATA LAKE :-
A data lake can be created and managed using a number of capabilities and services provided by Amazon Web Services (AWS). Organizations can store all of their structured and unstructured data in a data lake, which is a central repository that works at any scale. Here are a few characteristics of the Amazon data lake :
Amazon S3 :- The main storage service for constructing data lakes is Amazon S3 (Simple Storage Service). It offers scalable object storage for data of any size and kind.
AWS Glue :- Data transfer between data stores is simple with the help of AWS Glue, a fully-managed extract, transform, and load (ETL) service. Additionally, it has the ability to automatically find and collect metadata related to your data.
AWS Lambda Functions:- In AWS Data Lake, Lambda functions can play a crucial role in automating and enhancing data processing workflows. Data Transformation : Lambda functions can be used to transform data as it is ingested into the Data Lake. Event Processing where Lambda will do automatic processing of data reducing the need for manual intervention.The pay as you go model will help in optimize the costs in Data Lake architecture and Lambda being serverless, can automatically scale up and down to varying workloads.
Amazon Athena :- Data in Amazon S3 may be analyzed using conventional SQL thanks to Amazon Athena, an interactive query service. As there is no infrastructure to set up, it is serverless.
Amazon EMR :- Processing massive volumes of data using distributed frameworks like Hadoop, Spark, and Presto is simple with Amazon EMR (Elastic MapReduce), a fully-managed service.
AWS Lake Formation :- You may quickly and securely create a data lake using the AWS Lake Creation service. Data transformation, data access controls, and categorization are just a few of the functions it offers.
AWS Glue DataBrew :- It’s simple to clean and normalize data for analysis using Amazon Glue DataBrew, a visual tool for data preparation.
Amazon Redshift :- A cloud data warehouse called Amazon Redshift makes it simple to analyze sizable amounts of structured data. It is compatible with additional AWS services like Amazon EMR and AWS Glue.
Amazon Kinesis :- The platform for streaming data on AWS is called Amazon Kinesis. You can use it to gather, process, and analyze streaming real-time data from a variety of sources.
Amazon QuickSight :- For your data lake, it is simple to generate visualisations and dashboards using Amazon QuickSight, a cloud-based business intelligence solution.
These are just a handful of the numerous Amazon data lake functionalities that are offered. They offer an extensive collection of tools for creating, maintaining, and analyzing data lakes at scale when used collectively.
Advantages of AWS Data Lakes :-
You can safely store, examine, and share massive volumes of data at scale with the fully managed service provided by AWS Data Lake. Using AWS Data Lake has a number of benefits, such as:
Scalability :- Petabytes of data may be handled by AWS Data Lake, which scales itself as your data increases.
Cost-effective :- It’s a cost-effective method for handling massive volumes of data because you only pay for the storage and computing resources you really use.
Security :- To protect your data, Amazon Data Lake offers a number of strong security features, including access control, auditing, and encryption both in transit and at rest.
Flexible :- You can choose the appropriate tool for the task by utilizing AWS Data Lake, which supports a number of data formats, including structured, semi-structured, and unstructured data.
Integrations :- You may utilize the ideal tool for the job with Amazon Data Lake since it supports a wide range of data formats, including structured, semi-structured, and unstructured data.
Analytics :- Many analytics tools, like Amazon Athena, Amazon EMR, and Amazon Redshift, are available through AWS Data Lake, making it simple to query and analyze your data.
Collaboration :- When working with coworkers and business partners, Amazon Data Lake makes it simple to securely share data with other users and applications.
AWS Data Lake Architecture :-
Organizations can store, manage, and analyze vast amounts of data from several sources using the scalable and secure AWS Data Lake data repository. The architecture of AWS Data Lake typically consists of the following components:

Data Sources :-
Data from several sources, including databases, applications, IoT devices, and social media platforms, can be ingested by AWS Data Lake. These data sources could be local or online.
Data Ingestion :-
AWS offers a number of services, including Amazon Kinesis, AWS Glue, and AWS Data Pipeline, for importing data into the Data Lake.
Data Storage :-
Amazon S3, Amazon EBS, and Amazon Glacier are just a few of the storage solutions that AWS Data Lake provides. With its limitless scalability, superior durability, and affordable pricing, Amazon S3 is the most widely used storage option.
Data Catalog :-
Users may find, comprehend, and manage the data that is stored in the Data Lake using the data catalogue that is offered by AWS Glue. Column names, table definitions, and other metadata are included in the data catalogue.
Data Processing :-
For processing data kept in the Data Lake, AWS offers a number of services like Amazon EMR, AWS Glue, and Amazon Athena. These services can be utilized for activities including data analysis, data cleansing, and data transformation.
Data Visualization :-
AWS offers a number of services for displaying data from the Data Lake, including Amazon QuickSight, which enables customers to build interactive dashboards and reports.
Security and Governance :-
For the protection of the privacy, accuracy, and accessibility of the data kept in the Data Lake, AWS offers a number of security and governance capabilities. Encryption, access management, and audit recording are some of these characteristics.
All things considered, the design of AWS Data Lake offers a highly scalable, safe, and economical option for storing and processing huge volumes of data.
limitations of AWS Data Lakes :-
AWS Data Lake has a lot of benefits, but there are a few potential drawbacks to take into account as well:
Complexity :- It can be difficult to set up and administer an Amazon Data Lake, especially if you are unfamiliar with the AWS ecosystem.
Cost :- While AWS Data Lake can be inexpensive, if you plan to store a lot of data or make a lot of queries, this cost-effectiveness may not last.
Expertise :- You might need to have knowledge of data engineering, data architecture, and data analytics to make the most of AWS Data Lake.
Integration :- While many Amazon services are compatible with AWS Data Lake, not all third-party programs or data sources may be compatible with it.
Latency :- There can be some latency while accessing and searching your data, depending on how you configure your AWS Data Lake.
Maintenance :- Amazon Data Lake needs regular maintenance, just like any other IT system, to guarantee optimum performance and security. It may take a lot of time and resources to do this.
When deciding whether to use AWS Data Lake for your particular use case, it is crucial to balance these potential drawbacks with the advantages of doing so.
Conclusion :-
In general, AWS data lake offers a wide range of advantages, such as streamlined data administration, enhanced data quality and accessibility, accelerated time to insights, and cost savings. But setting up and maintaining an AWS data lake requires knowledge of data management and AWS services, so it’s crucial to carefully plan and design the architecture to make sure it satisfies the organization’s unique requirements.
Migration Readiness Assessment (MRA) Tool Overview
Are you ready for the Cloud?
A successful cloud migration begins with a detailed analysis of goals, business plans, and resources currently used, with the initial purpose of gaining a clear understanding of the starting point, discovering the gaps to be filled, and developing a strong business case. It is in this exact context that our Accelerated Cloud Exploration (ACE) program comes into play: as an Advanced AWS Consulting Partner, a team of specialists from Ankercloud guides our customers through a complete assessment phase covering all these points to make a well-informed decision on whether and how to move to the cloud.
Ankercloud’s expertise is combined with a variety of professional tools offered by AWS, starting with the Migration Readiness Assessment (MRA), which represents the first hands-on activity to embark on the Cloud Exploration journey. A customized roadmap is built to define the successive actions to be taken.
What is the MRA tool?
The Migration Readiness Assessment (MRA) tool is used to assess the customer’s strengths and weaknesses following the 6 areas of the AWS Cloud Adoption Framework: Business, Platform, People, Governance, Operations, and Security. It evaluates existing skills and set-up on a scale from 1 to 5, highlighting the readiness level and giving an overall score for each main focus area and related subtopics.
How does it work?
The process requires you to answer 80+ questions and is typically fulfilled in a 1-day workshop, but it can also take several days. Due to the difficulty of the topics and the depth of the analysis, it is important that the customer’s team and the experts from Ankercloud work well together to finish the tasks.
What is the outcome?
After data collection, Ankercloud generates a full report that includes charts, scores, and data visualization. Together with the customer, we can look at the results and determine which areas need more work and which are already ready to move to the cloud.
But the MRA workshop is just the beginning.
To finish the business case, the Total Cost of Ownership (TCO) must be calculated, and it may be necessary to evaluate how the on-premise resources are set up.
The AWS Migration Evaluator and Migration Portfolio Assessment are suitable tools to fulfill the missing analysis and guide our customers towards the completion of the ACE Program, ready for a PoC implementation. Based on the MRA results as well as the outcome of the other components of ACE, our customers have full visibility of the strengths and weaknesses of a potential migration to the cloud and can make an informed and confident decision if and how to migrate.
Further learning…
Are you willing to discover what the AWS cloud can offer but unsure what the best way to start is? Want to know more about MRA and the other tools available to complete the assessment phase, as well as our Accelerated Cloud Exploration program? Don’t hesitate to reach out to us, we will be more than happy to solve all your doubts and give support for getting started with AWS cloud technologies.
Migration of Servers from Digital Ocean to AWS
Hello, When starting the process of migrating servers from Digital Ocean to AWS. I choose AWS Application Migration Service after carefully reading the documentation to decide which AWS service to use for the migration.
Why I Selected the AWS Application Migration Service:
AWS Application Migration Service (MGN) is a highly automated lift-and-shift (rehost) solution that simplifies, expedites, and reduces the cost of migrating applications to AWS. It enables companies to lift-and-shift a large number of physical, virtual, or cloud servers without compatibility issues, performance disruption, or long cutover windows.
As per the Requirement of customers they want to clone their complete server as it is on AWS, so as MGN gives us Lift and Shift of everything we have on our servers I decide to go with it.
Moving to the Steps of Migration:
The Servers which are in the digital ocean they all are in the public subnet, so I have asked for the access of all the servers which I want to migrate. (If you don’t have access of source servers then share the replication agent download and installation link with your client)
1. Creating User:
I. As the First step you have to Login to AWS console-> go to the IAM-> create new user: MGNUSER->and add the permissions -> AWSApplicationMigrationAgentPolicy -> click create user
II. Then generate Access keys and Secret keys for the MGNUSER (we need these keys for creating source server in MGN.
2. Download and Installation of Replication Agent on Source
I. Navigate to Application Migration Console-> Go to Source Server-> Add server-> Select your OS type-> Enter Your MGNUSER Access key and Secret Key.

I. Then copy the replication download link and run it on source server.
II. Once downloaded, copy the replication installation agent link and run it on your source server.
III. It will take some time for the command line to appear on your source server (depending typically on network bandwidth and network connectivity between Digital Ocean and AWS). Keep your terminal open throughout this operation.
IV. Once the Process completed on your source server you can close your source terminal.
V. Note: The AWS Application Migration service did not support Ubuntu version 21
1. Configuration on AWS
I. Go to Application Migration Service in AWS It is possible to observe that the new server has been built and is currently synchronizing (again, it is not required that you remain logged into your AWS account and continuously monitor the developments).
II. The continuing Synchronous procedure will not be impacted by your network connectivity loss or logging out of your AWS account.
III. The Synchronous process will be completed and marked as being ready for testing (the Synchronous process takes hours to complete depending on the size of data on source).
2. Launch Test Instance
I. Select your server-> go to the Launch Configuration-> mention the Network Configurations-> size of instance-> its VPC-> subnet
II. Click on Launch Test instance

III. It will create the job Id which will track the complete process.

IV. Once the EC2 instance launched successfully you can see that instance in your console.
V. Test the Instance. If everything is good then mark it as revert for cutover.
Note: During the process the changes which you are performing on source that is digital ocean server will not automatically add to the Launched EC2 instance.
Example:
If I have 10 files on sources at the time of launching the instance and the instance launched in AWS.
Now that I’ve made changes on the digital ocean server, I’ve added one file there, making a total of 11 files.
But that will not reflect on my EC2 for that updated changes we have to Revert the instance back to testing and then Launch the test instance using same Launch template.
VI. Once test done Mark it as Ready for cutover.
1. Launch Cutover instance
Points to be noted before cutover:
Make a plan for cutover it should be in non-business hours
Don’t shutdown your source server before finalizing the cutover.
I. Select the Launch Template configuration, do the configurations.
II. Select Launch cutover Instances
III. The cutover job will be created, See the job progress
IV. Once the Instance Successfully Launched.
Note: It will Terminate your already Launched test instance and will Launch new Instance.
V. Click on Finalize Cutover
VI. Now you successfully migrated the Server from Digital Ocean to AWS
VII. Terminate your Digital Ocean server and start using the newly Migrated AWS Server.
Conclusion
If you have any questions about moving a server from Digital Ocean to AWS, I hope this blog will help. Check your OS version before moving, keep tabs on source updates before cutting the switch, choose a schedule for the switch, and follow the instructions provided. I hope this will save you time, money, and risk.
The Rise of Serverless Computing
Small and medium businesses including large enterprises are evolving rapidly leveraging Serverless computing. Even companies like Amazon, Google and Microsoft have dedicated branding for Serverless Computing, indicating this is the next big thing in the world of cloud computing.
But what exactly is Serverless Computing?
Serverless computing is a cloud-based service where a cloud provider manages the server. The cloud provider dynamically allots compute storage and resources as needed to execute each line of code. Importantly, Serverless computing is event-driven, meaning developers can create states as I/O requests that are received and then destroyed in compute instances. The process is 100% automated and does not require human interaction and maintenance the way a traditional server would need. This makes Serverless computing an efficient, affordable, and resource-effective way to build and use applications.
Amazon CTO Werner Vogels on Invent keynote, pressed about the trajectory of serverless computing, particularly with enterprises. He said..
“The whole notion of only having to build business logic and not think about anything else really drives the evolution of Serverless Computing.”
With the serverless computing model, organizations pay for the amount of time and memory an application’s code takes to perform the tasks it needs to. Amazon calls this measurement gigabyte-seconds.
Serverless computing services are available in two ways: Backend-as-a-Service (BaaS) and Function-as-a-Service (FaaS). Some providers offer database and storage services to customers or BaaS, while others offer functions without storing application data as the service.
There are many serverless providers in the market. However, here are the best companies in the market:
AWS: Athena, Lambda, Step Function, DynamoDB, Aurora, API Gateway, etc
Microsoft Azure: Azure Functions
GCP: Cloud Functions, App Engine, Cloud Run etc.
Now, we hope you understand the serverless concept. Let us understand how it is helping companies across the globe, below are the benefits.
Key business benefits of Serverless Computing:-
- Quick Deployment:- Adopting a serverless architecture removes a lot of complexity and delay and helps teams deploy products quickly.
- Easy Scalability:- The serverless model also boosts a company’s ability to quickly scale services. Because they’re not limited by server capacity and they can scale services up or down depending on business needs or ambitions.
- Greater Cost-efficiency:- As companies don’t have to pay for idle resources, teams can quickly adjust spending according to service needs.
- Improved Flexibility:- It’s easier to begin the implementation of an app serverless than it is with traditional methods. Because of that, going serverless means you can innovate faster as well, It’s also easier to pivot in situations where you need to restructure.
- Pay-as-you-go Model:- It means that the consumers are only going to be charged the number of times their piece of code runs on a serverless service.
Let’s understand how serverless computing can have an impact on business growth.
- With Serverless computing architecture, enterprises can enhance scalability, enable pay-per-use capabilities and lower costs.
- Serverless computing has capabilities to eliminate infrastructure management tasks, reduce operating system maintenance costs, and encourage capacity provisioning and patching. Besides, the rising focus of companies towards serverless infrastructure is likely to offer lucrative opportunities in the market.
- Help improve operations by decreasing downtime and increasing overall efficiency saving them time and money in the process.
- Serverless computing will allow your enterprise to embrace digital transformation and optimize the opportunities created by the modernization of the application and infrastructure stack that will usher in new modes of automation, management, DevOps, and security.
Conclusion:
To sum up, Serverless Computing is the future of cloud computing. It provides the companies with the capability to be more agile, cost-effective and increase their overall operational efficiency. Serverless computing could be one of the most exciting developments of the 21st century. For those looking to build event-based apps quickly and efficiently, serverless computing is the way to conserve resources, increase efficiency, and boost productivity.
If this is the need of the hour for your business, we are here to help! Write to us at info@ankercloud.com and we will get back to you!

Saasification and Cloud Migration for vitagroup: a key player in the highly-regulated German Healthcare sector

Enhancing DDoS Protection with Extended IP Block Duration Using AWS WAF Rate-Based Rules
.png)
Smart Risk Assessment: Bitech’s AI-Driven Solution for Property Insurance

Streamlining CI/CD: A Seamless Journey from Bitbucket to Elastic Beanstalk with AWS CodePipeline
.png)
Setting Up Google Cloud Account and Migrating Critical Applications for Rakuten India

Migration to Cloud and Setting Up of Analytics along With Managed Services
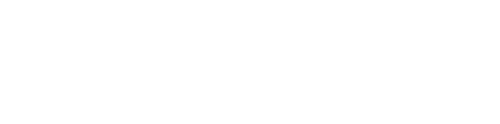
gocomo Migrates Social Data Platform to AWS for Performance and Scalability with Ankercloud
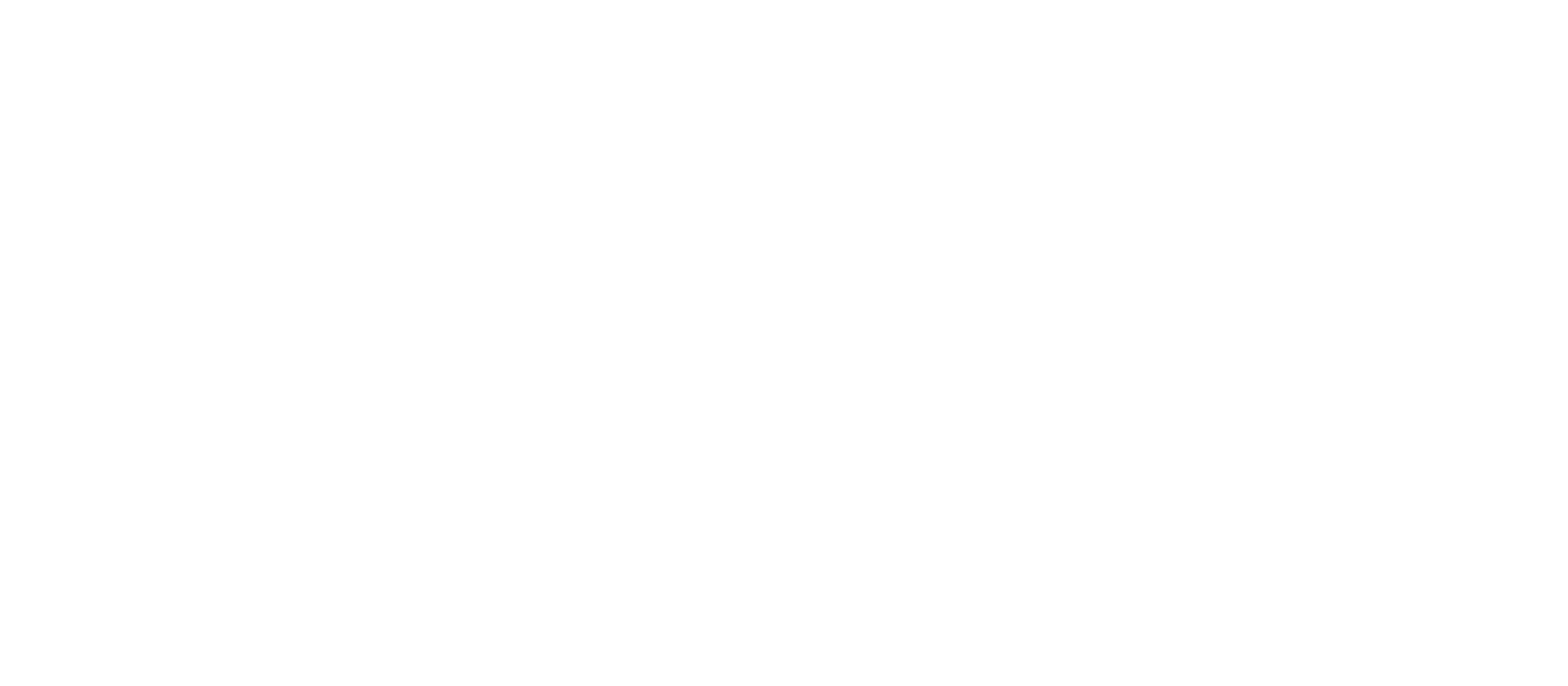
Benchmarking AWS performance to run environmental simulations over Belgium
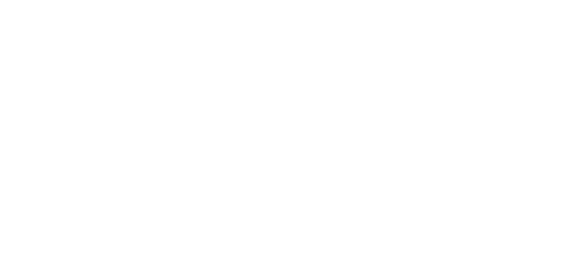
Model development for Image Object Classification and OCR analysis for mining industry

Achieving Cost Optimization, Security, and Compliance: Ankercloud's AWS CloudOps Solutions for Federmeister
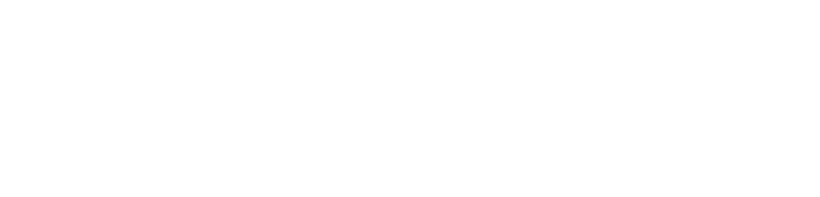
Replication of On-premise Infrastructure into AWS Cloud on Docker Swarm platform
Please Type Other Keywords
The Ankercloud Team loves to listen
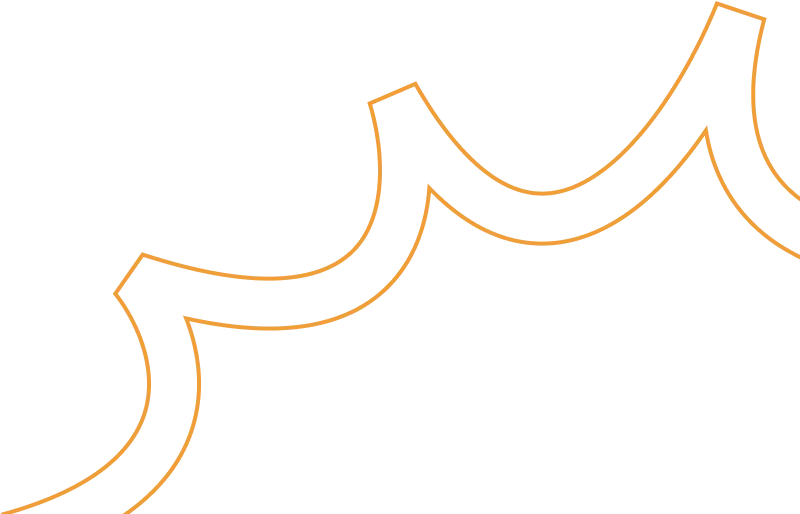
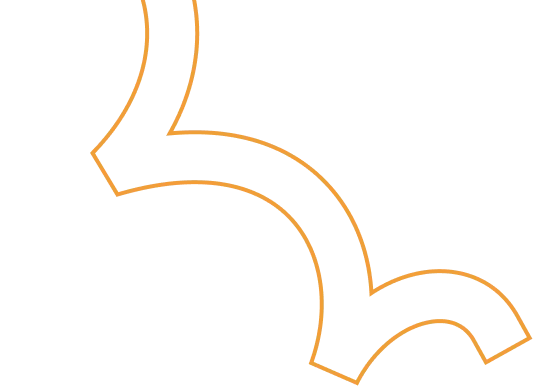