Resources
The latest industry news, interviews, technologies and resources.
DORA Metrics for DevOps Performance Tracking
INTRODUCTION TO DORA METRICS:
This blog is to explain the DevOps Research and Assessment capabilities to understand delivery and operational performance for better organizational performance.
DORA — DORA (DevOps Research and Assessment) metrics help us to measure the DevOps performance if there are low or elite performers. The four metrics used are deployment frequency (DF), lead time for changes (LT), mean time to recovery (MTTR), and change failure rate (CFR).
The four essentials of DORA metrics:
- Deployment frequency
- Lead time for changes
- Mean time to recovery
- Change failure rate
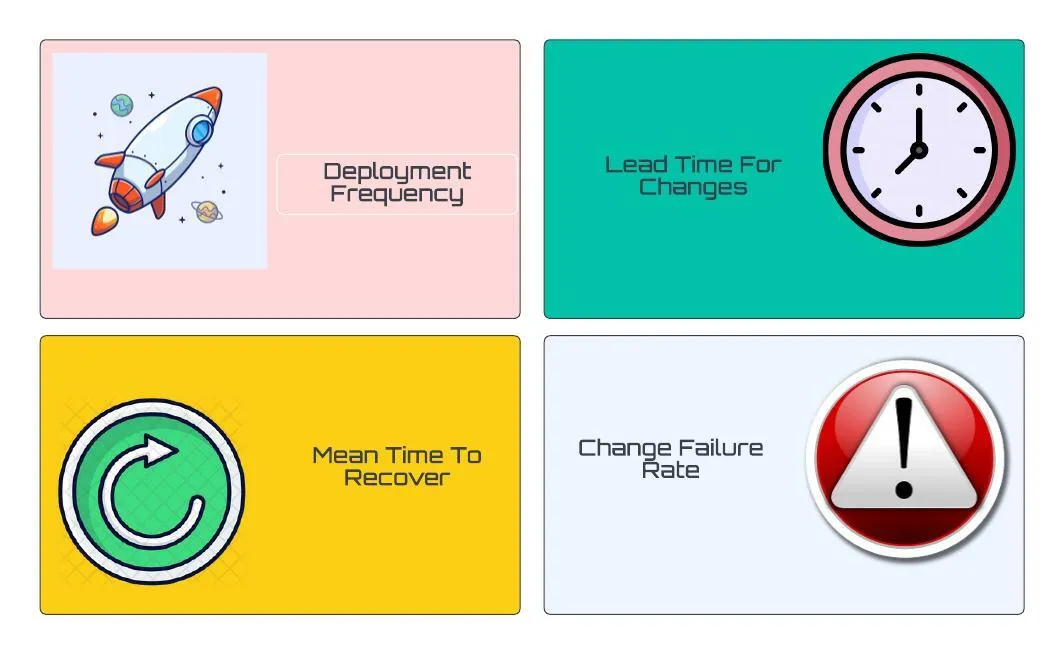
Deployment Frequency :
Deploy frequency measures how often you deploy changes to a given target environment. Along with Change lead time, Deploy frequency is a measure of speed.
Deployment Frequency also provides us with Batch Size Breakdowns,
Allowing you to filter the code changes based on Small batch, Medium, Large, and Gigantic batch sizes.
- Small — usually 1 pull request, 1–10 commits, and a few hundred lines of code changed
- Medium — usually 1–2 pull requests, 10–30 commits, and many hundreds of lines of code changed
- Large — usually 2–4 pull requests, 20–40 commits, and many hundreds of lines of code changed
- Gigantic — usually 4 or more pull requests or 30 or more commits or many thousands of lines of code changed.
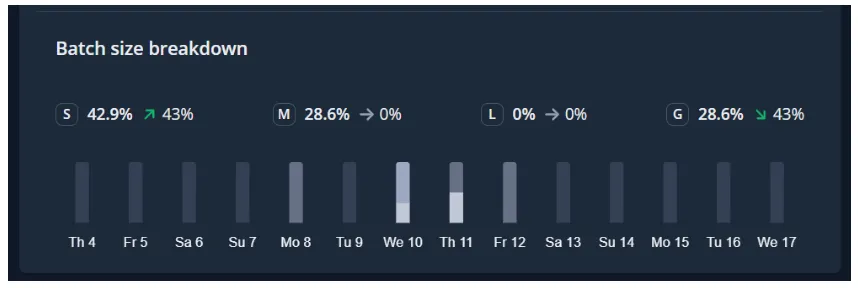
Lead Time for Change:
Change lead time measures the time it takes for a change to go from its initial start of coding to being deployed in its target environment. Like Deploy frequency, Change lead time is a measure of speed (whereas Change failure rate and MTTR are measures of quality or stability).
In addition to the Lead Time for Change, Sleuth provided us with a detailed breakdown of how much time your teams, on average, are spending.
- Coding — the time spent from the first commit (or the time spent from the first transition of an issue to an “in-progress state) to when a pull request is opened
- Review lag time — the time spent between a pull request being opened and the first review
- Review time — the time spent from the first review to the pull request being merged
- Deploying — the time spent from pull request merge to deployment
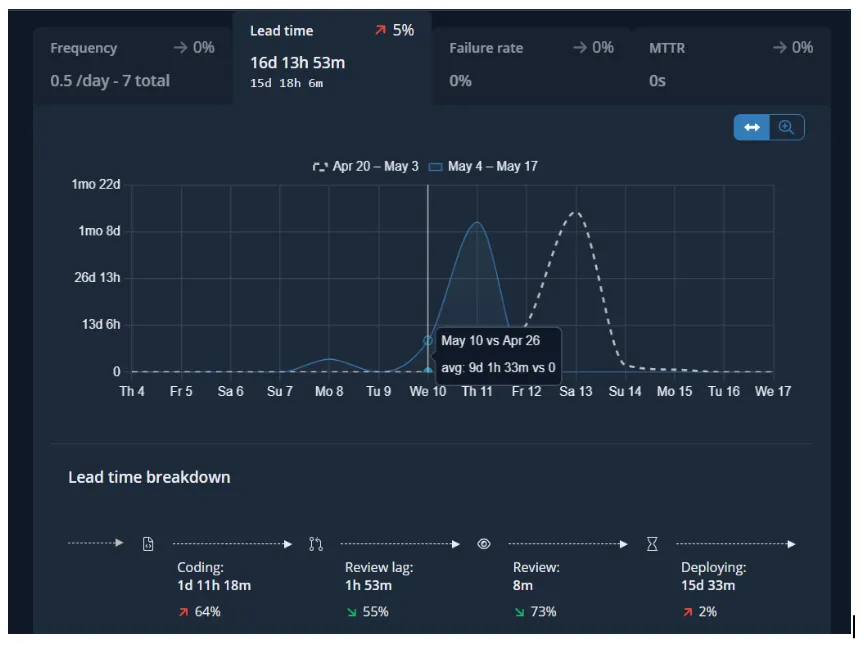
Mean Time to Recovery:
Change failure rate measures the percentage of deployed changes that cause their target environments to end up in a state of failure. Along with MTTR, Change failure rate is a measure of the quality, or stability of your software delivery capability.

Change Failure Rate
Measures the quality and stability while deployment frequency and Lead Time for Changes don’t indicate the quality of the software but just the velocity of the delivery.
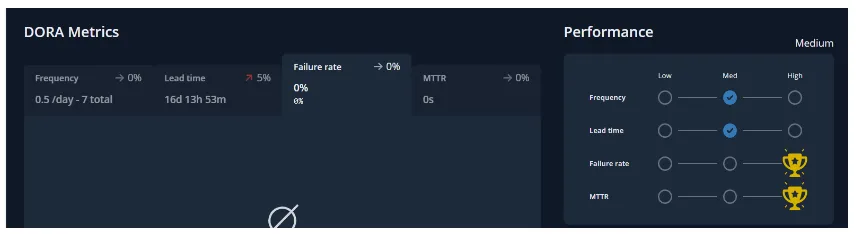
Here’s a table of how DORA metrics are calculated depending on the deployment that occurred, coding and review time, time is taken to restore from an incident or a failure, and failure rate that occurred due to the deployments.
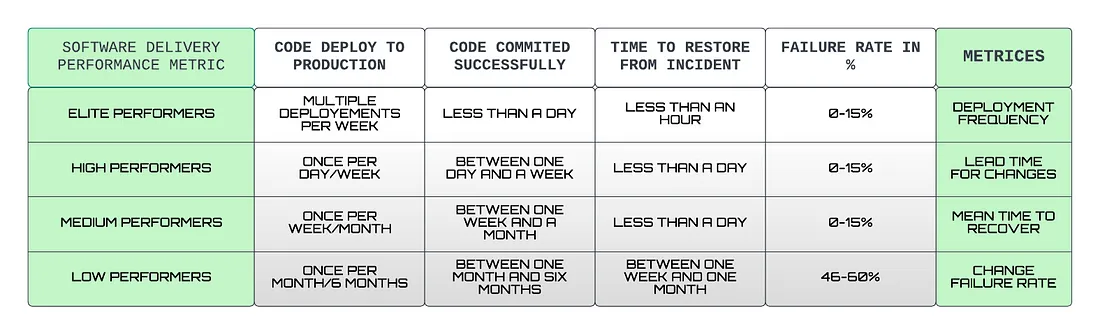
Apart from the four metrics of DORA, there is a fifth one, Reliability, happens to be most important when it comes to operational performance which brings together DevOps and SRE teams to build us a better infrastructure and software. The Reliability metrics is a great way to showcase a team’s overall software delivery performance.
Become an Elite :
According to the most recent State of DevOps report, elite performers have recently grown to now represent 20% of survey respondents. High performers represent 23%, medium performers represent 44%, and low performers only represent 12%.
CONCLUSION :
DORA metrics are a great way to measure the performance of your software development and deployment practices. DORA metrics can help organizations to measure software delivery and stability to a team’s improvement, which also decreases the difficulties and allows for quicker, higher quality software delivery.
DevOps Trends: CI/CD Automation
CI/CD (Continuous Integration/Continuous Delivery) automation is a crucial aspect of DevOps practices and has been gaining significant attention in recent years. By automating the CI/CD pipeline, organizations can accelerate software delivery, improve code quality, and enhance collaboration between development and operations teams. Here are some notable trends in CI/CD automation:
- Shift-Left Testing: Shift-left testing emphasizes early and continuous testing throughout the software development lifecycle, starting from the earliest stages of development. By integrating testing into the CI/CD pipeline and automating the testing process, organizations can identify and address issues more quickly, reducing the risk of defects reaching production.
- Infrastructure as Code (IaC): Infrastructure as Code is a practice that enables the automation and management of infrastructure resources using code. With IaC, infrastructure configurations can be version-controlled, tested, and deployed alongside application code. CI/CD automation tools integrate with IaC frameworks such as Terraform or AWS CloudFormation to provision and manage infrastructure resources in a consistent and repeatable manner.
- Cloud-Native CI/CD: As organizations increasingly adopt cloud computing and containerization technologies, CI/CD pipelines are evolving to support cloud-native applications. Tools like Kubernetes and Docker are commonly used to build, deploy, and orchestrate containerized applications. CI/CD automation platforms are adapting to support the unique requirements of cloud-native environments, enabling seamless integration with container registries, orchestrators, and serverless platforms.
- Machine Learning/AI in CI/CD: Machine learning and AI techniques are being applied to CI/CD automation to optimize various aspects of the software delivery process. For example, AI-based algorithms can analyze code quality, identify patterns, and provide recommendations for improvements. Machine learning models can also be used to predict and detect anomalies in CI/CD pipelines, enabling proactive identification of potential issues.
- Low-Code/No-Code CI/CD: The rise of low-code/no-code development platforms has extended to CI/CD automation as well. These platforms provide visual interfaces and pre-built integrations that simplify the setup and configuration of CI/CD pipelines, reducing the need for extensive coding or scripting. Low-code/no-code CI/CD tools empower non-technical stakeholders to participate in the automation process and accelerate the delivery of applications.
Benefit of CI/CD: -
· Increased delivery speed & cooperation
· Instantaneous feedback
· Simple to maintain & Reliable
Components of a CI/CD Pipeline: -
a. Jenkins Pipeline: Jenkins Pipeline is a powerful and flexible way to define your continuous integration and continuous delivery (CI/CD) workflows in Jenkins. It allows you to define your build, test, and deployment stages as code, providing a consistent and repeatable process for your software development lifecycle. Jenkins Pipeline supports two syntaxes: Declarative Pipeline and Scripted Pipeline.
i. Declarative Pipeline: Declarative Pipeline provides a more structured and opinionated syntax for defining pipelines. It is recommended for most use cases as it offers simplicity and readability. Here’s an example of a simple Declarative Pipeline:
pipeline { agent any
stages { stage(‘Build’) { steps { // Perform the build steps here } }
stage(‘Test’) { steps { // Run your tests here } }
stage(‘Deploy’) { steps { // Deploy your application here }}}}
In this example, the pipeline has three stages: “Build,” “Test,” and “Deploy.” Each stage contains the necessary steps to be executed.
ii. Scripted Pipeline: Scripted Pipeline provides a more flexible and programmatic way to define your pipelines using Groovy scripting. It allows you to have greater control over the execution flow and provides more advanced features. Here’s an example of a simple Scripted Pipeline:
node {
stage(‘Build’) { // Perform the build steps here}
stage(‘Test’) { // Run your tests here }
stage(‘Deploy’) { // Deploy your application here }}
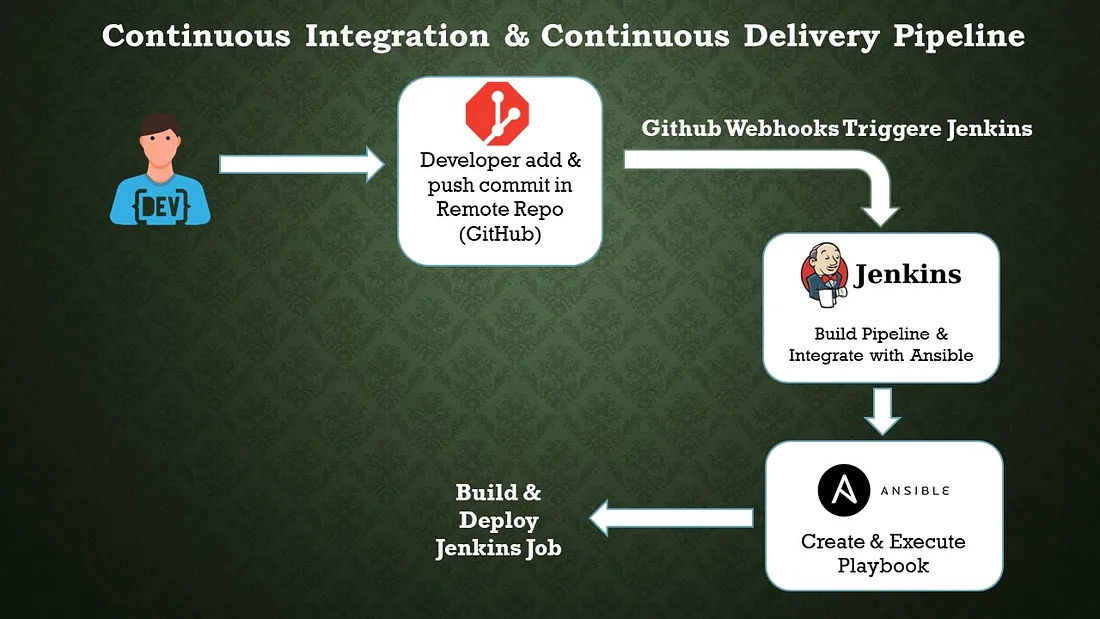
b. Configuration Management Tool: Ansible
Ansible is an open-source configuration management tool that automates the deployment, orchestration, and management of software applications and infrastructure. It is designed to be simple, agentless, and easy to use, making it popular among system administrators and DevOps teams. Here are some key features and concepts related to Ansible:
i. Agentless: Ansible does not require any agents or additional software to be installed on the target systems. It uses SSH (Secure Shell) and Python to communicate with remote hosts, which simplifies the setup process and reduces the overhead on managed systems.
ii. Declarative Language: Ansible uses a YAML-based language called Ansible Playbooks to define configurations and automate tasks. Playbooks are human-readable and describe the desired state of the systems. This declarative approach allows for idempotent execution, where running the same playbook multiple times produces consistent results.
iii. Inventory: Ansible uses an inventory file to define the hosts or systems it manages. The inventory can be a static file or generated dynamically from various sources, such as cloud providers or external scripts. It allows you to organize hosts into groups and apply different configurations to specific groups or individual hosts.
iv. Modules: Ansible comes with a wide range of built-in modules that perform specific tasks, such as managing packages, configuring services, manipulating files, or executing commands. Modules are written in Python and can be extended or customized to meet specific requirements.
v. Playbooks: Playbooks are the heart of Ansible. They are YAML files that define a set of tasks to be executed on remote hosts. Playbooks specify the desired state of the systems, and Ansible takes care of bringing them into that state. Playbooks can include variables, conditionals, loops, and handlers to perform complex configuration management.
vi. Idempotency: Ansible’s idempotent nature ensures that running the same playbook multiple times does not cause unintended changes. If a system is already in the desired state, Ansible skips the corresponding tasks, resulting in a consistent and reliable configuration management process.
vii. Ad-hoc Commands: Ansible allows you to execute ad-hoc commands directly on remote hosts without the need for writing a playbook. This feature is useful for quick troubleshooting, one-time tasks, or running simple commands across multiple systems simultaneously.
viii. Ansible Galaxy: Ansible Galaxy is a hub for sharing and discovering Ansible roles. Roles provide a way to organize and reuse playbook logic, making it easier to manage complex configurations. Ansible Galaxy allows you to find pre-built roles contributed by the community, helping you accelerate your automation efforts.
Conclusion:
Code quality is increased and changes are provided rapidly with CI/CD automation. The automation technique has a very good quality, bug-free, and quicker fault isolation impact. We completed every step of the automation process, including create, build, test, and deliver. Process automation is necessary for software development.
Introducing ACE — our Accelerated Cloud Exploration program!
Do you have too much data to handle and analyze?
Are your IT budgets maxed out and you are unsure if Cloud is a good alternative?
Are you uncertain if Cloud aligns with your security requirements and can align with business processes?
When it comes to migrating to the cloud there are many different scenarios and challenges our customers need to assess and tackle. One of the above questions can be the trigger moment to consider migrating to or modernizing within the cloud. But what does migration imply?
When we talk about migration it could be the traditional case of a full IT migration from on-prem or one cloud provider to the other, but it can also mean bringing a large workload — like a whole Machine Learning application — into an existing infrastructure on the cloud. We also talk about a migration case when a customer is planning to add a new component to existing infrastructure or is modernizing and reshaping their cloud infrastructure.
Since there are so many possible reasons to consider choosing Cloud and every requirement and use case is unique, we have developed a new program — the Accelerated Cloud Exploration (ACE) — to help our customers assess their status quo and get full visibility on relevant stakeholders, timelines, a detailed analysis of Cost of Ownership (TCO) along with a Testbed/Sandbox when considering migrating to the cloud.
What is it?
ACE contains the components of the AWS MAP Assess phase and combines them with the substantial migration expertise and experience of Ankercloud as well as the speed and agility that we can provide through the strength of our global team.
How does it work?
The program runs in a 4–6-week time frame in which we conduct several workshops, deep dive sessions and prepare testbeds/Sandboxes together with our customers, and create a detailed report which provides you with all aspects of cloud adoption for your needs.
What is Included?
· Migration Readiness Assessment — The first workshop focuses on examining the scope and targets of a potential migration as well as shedding light on the current platform setup, governance, and security requirements by analyzing our customers’ readiness/adoption factors.
· Discovery Workshop — Once we have the business, product, and organizational alignment, we move our focus to the current technology inventory like the existing application stack and databases to then start mapping the right services and infrastructure on AWS.
· Migration Patterns and Architectures — After the Discovery Workshops, we built an exact AWS architecture that would suit your needs. We create the exact architectural diagrams, configurations and systems that enable them to adopt new cloud services or replace existing infrastructure with AWS.
· Total Cost of Ownership (TCO) Analysis –Using this architecture and understanding of your utilization, we develop an investment plan and ROI analysis for the next 36 months by accounting for post-migration AWS costs, saving costs from alternative options, and providing the correct infrastructure sizing and configurations.
· Proof of Concept (POC) — While the previous phases of this program focus on helping you get complete visibility of all facets of cloud adoption, we go one step further to help you get a direct hands-on taste of it. Within ACE, we also include a PoC to provide our customers with a sandbox environment or application on AWS to experience the advantages of a migration firsthand and get their developers a “look and feel” of their post-migration infrastructure.
· Carbon Emission Calculation — In every MAP Assess project we make use of the AWS Carbon Footprint tool which allows us to include detailed calculations and comparisons of on-prem vs. AWS CO2 emissions into the report and highlight CO2 savings for the customer.
How Much Does ACE Cost?
Depending on your current and future IT Infrastructure plans, we can provide ACE program free of charge (i.e. 100% discount/ funding).
Furthermore, after this program there is further incentivization in working with us — any follow-up activities that you would like to work on with us, for example — database and server migration, application migration, and creation of various IT environments are discounted by 50%.
And there’s more — If you do choose to migrate your workloads to AWS after the ACE program, you get 25% off on your AWS bills towards any new migrated workload for the first 36 months.
Sounds Interesting?
Our ACE Program, in collaboration with AWS, is the perfect way to start exploring the cloud as the next step in your IT or Product expansion and scaling plans. And you can now make that decision with an experienced external partner with potentially zero costs. If that sounds like an exciting proposition reach out to us at cloudengagement@ankercloud.com
How to build a Software-as-a-Service (SaaS) product on AWS
More and more companies operating in the IT sector are born with, have switched to, or are evaluating the Software-as-a-Service (SaaS) business model as an effective way to deliver their services to customers. SaaS in the cloud is the perfect solution to leverage all the available modern tools and automated processes, but how much do you know about the optimal way to build these products on AWS?
The problem
Let’s say that your company is interested in managing a SaaS product on AWS, but you are unsure how you should approach the problem or how to start implementing a new feature that needs to be integrated with the offer. Whether you are:
- Thinking about adopting a SaaS model
- Planning to onboard a lot of new customers
- Already using SaaS, on AWS or on another platform
- Working on new license-based solutions
- Looking to modernize your whole setup or a specific part of it
- Interested in improving your DevOps pipeline
… we at Ankercloud think you could strongly benefit from the AWS SaaS Discovery Program.
The solution
Being a SaaS-certified partner and benefitting from tight cooperation with AWS, Ankercloud embarks you on a discovery journey with the aim of giving you full guidance for SaaS-related innovations, customized to your needs. That’s what the SaaS Discovery Program is all about: a period of time ranging from 2–4 weeks to be spent together, starting with technical deep dive workshops to align on your specific starting point and requirements, all the way into AWS architecture design, modernization discussions, TCO computation, best practices explanation, and much more — always suited to your business case.
But the good part does not end here: depending on your growth potential, we are able to provide the SaaS Discovery Program free of charge for you (i.e. 100% discount/funding).
High Potential use cases
The focus of the SaaS Discovery Program is always to accommodate your needs and concentrate on improving your weak points. Depending on your inputs, examples of common use cases can be:
- SaaS Design Decomposition
- Authentication and Access Management
- CI/CD Pipelines
- Database Multi-tenancy and Tenants isolation
- Security and Reliability
- SaaS DevOps
- Agility and Operations
But this list is non-exhaustive, and we at Ankercloud are always open to learning about your specific obstacles and understanding how we can support you. And here is our challenge for you: bring us your most critical SaaS-related issue, we will be happy to discuss it and bring all our deep technical knowledge to develop a solution together.
What about the outcome?
This program is intended to provide flexibility and visibility during the whole planning and discovery process. Therefore, once the program is completed, there is no obligation to further continue with the implementation of the developed solution on AWS: no commitment of any kind is in fact implied, as the name discovery suggests.
Several documents and deliverables will anyway help you in the decision-making process, giving full visibility to the planned solution. At the end of the program, Ankercloud will in fact provide you with a detailed technical report with an architecture diagram, a complete analysis of the AWS costs within an 18 months time horizon, and a full proposal to continue working together with the implementation, to give us the possibility of providing further hands-on support if needed.
Sounds interesting? Are you ready to start exploring new SaaS solutions and best practices?
Don’t hesitate to contact us at: cloudengagement@ankercloud.com
Let us guide you through the steps and check your eligibility for the SaaS Discovery Program.
The SaaS Growth Story
Software-as-a-service (SaaS) on Cloud
Software-as-a-service (SaaS) has been around since the early 2000s and is a cost-effective alternative to the traditional IT deployment where customers have to buy or build their own IT infrastructures, install the software themselves, configure the applications and employ an IT department to maintain it all.
SaaS offers a connection and subscription to IT services built on shared infrastructure via the cloud and deployed over the internet, rather than purchased and downloaded or installed locally.
With the continuous growth of cloud computing and the clear advantages of subscription-based services, it comes as no surprise that the software as a service market continues to expand rapidly. Many organisations are committed to purchasing SaaS solutions rather than buying and hosting software internally.
Furthermore, on a SaaS provider side, this software distribution model makes it possible even for small companies, to reach a broad range of customers, opening doors to new markets and geographies.
„SaaS remains the largest public cloud services market segment, forecasted to reach $176.6 billion in end-user spending in 2022. Gartner expects steady velocity within this segment as enterprises take multiple routes to market with SaaS, for example via cloud marketplaces, and continue to break up larger, monolithic applications into compostable parts for more efficient DevOps processes.” Source: Gartner (April 2022)
Characteristics of SaaS
SEAMLESSLY AVAILABLE & SCALABLE
Uptime and the ability to respond to continually changing requirements and workloads build the basis for any successful SaaS product. Cloud provides a broad range of capabilities like x and y that can be leveraged to align with the uptime requirements of SaaS environments. It also provides dynamic scaling mechanisms that allow for the alignment of tenant consumption with the actual load.
PAY-AS-YOU-GO PRICING
Continuously managing and optimising costs is essential for SaaS providers. With the elasticity of the Cloud, they are able to build SaaS solutions that are optimised to match the infrastructure of a multi-tenant load and its scaling requirements.
GLOBAL REACH
One big advantage of the SaaS model is fast access to new markets and geographies. The availability of the public Cloud in all the principal geographic regions allows for global reach and high availability due to multi-region set-ups.
SECURITY
SaaS solutions hosted in the cloud providers can be distributed over multiple servers scattered in multiple geographical locations and have automatic backups, ensuring an extremely high level of security.
INNOVATION
The breadth and depth of tools and services available on the Cloud can facilitate a faster time-to-market for SaaS providers. The pace of innovation in the Cloud also provides SaaS companies with new services and capabilities to enhance the features, cost, and management profile of their solutions.
Making the shift: from on-prem to SaaS-enabled solutions
SaaS turns the traditional model of software delivery on its head. Rather than purchasing licenses, paying an annual maintenance fee for upgrades and support, and running applications in-house, SaaS allows organizations to buy only the number of licenses they require as their need fluctuate.
For a SaaS provider, the shift from providing on-premises solutions to becoming a SaaS-based solution provider involves intense levels of continuous testing. This means, by becoming a SaaS provider, there needs to be a shift of understanding within the organization to transform from being a software provider to a service provider.
From an operational perspective, this requires new capabilities, such as meeting service level agreements, establishing real-time usage monitoring and billing capabilities, and meeting strict security requirements.
The robust infrastructure required to provide SaaS services 24×7 requires a substantial investment.
The business challenges are even greater, ranging from the dramatically lower margins provided by SaaS, to changes in cash flow and pricing models, to requirements for customer support.
With this in mind, once a decision is made to make the shift, it will be important to rigorously evaluate the different potential SaaS models and adopt an iterative deployment approach allowing for greater learning and flexibility during the course of the deployment. Software companies and their customers should periodically assess their overall SaaS roadmap to regularly check their progress against their strategic goals.
Accelerate your SaaS journey with Ankercloud
While the advantages of a cloud-based SaaS model are strong and allow a company to focus on its core goals of developing, delivering applications, and improving its customer experience, it is important to pay special attention to key components like infrastructure budget management, capacity management, and platform availability. This is where an experienced SaaS partner like Ankercloud can be the key to a successful SaaS adoption. We support our customers on their journey to develop a SaaS model on AWS with a consolidated approach, years of experience, and deep AWS knowledge.
Curious? Reach out to us at cloudengagement@ankercloud.com
The Rise of Serverless Computing
Small and medium businesses including large enterprises are evolving rapidly leveraging Serverless computing. Even companies like Amazon, Google and Microsoft have dedicated branding for Serverless Computing, indicating this is the next big thing in the world of cloud computing.
But what exactly is Serverless Computing?
Serverless computing is a cloud-based service where a cloud provider manages the server. The cloud provider dynamically allots compute storage and resources as needed to execute each line of code. Importantly, Serverless computing is event-driven, meaning developers can create states as I/O requests that are received and then destroyed in compute instances. The process is 100% automated and does not require human interaction and maintenance the way a traditional server would need. This makes Serverless computing an efficient, affordable, and resource-effective way to build and use applications.
Amazon CTO Werner Vogels on Invent keynote, pressed about the trajectory of serverless computing, particularly with enterprises. He said..
“The whole notion of only having to build business logic and not think about anything else really drives the evolution of Serverless Computing.”
With the serverless computing model, organizations pay for the amount of time and memory an application’s code takes to perform the tasks it needs to. Amazon calls this measurement gigabyte-seconds.
Serverless computing services are available in two ways: Backend-as-a-Service (BaaS) and Function-as-a-Service (FaaS). Some providers offer database and storage services to customers or BaaS, while others offer functions without storing application data as the service.
There are many serverless providers in the market. However, here are the best companies in the market:
AWS: Athena, Lambda, Step Function, DynamoDB, Aurora, API Gateway, etc
Microsoft Azure: Azure Functions
GCP: Cloud Functions, App Engine, Cloud Run etc.
Now, we hope you understand the serverless concept. Let us understand how it is helping companies across the globe, below are the benefits.
Key business benefits of Serverless Computing:-
- Quick Deployment:- Adopting a serverless architecture removes a lot of complexity and delay and helps teams deploy products quickly.
- Easy Scalability:- The serverless model also boosts a company’s ability to quickly scale services. Because they’re not limited by server capacity and they can scale services up or down depending on business needs or ambitions.
- Greater Cost-efficiency:- As companies don’t have to pay for idle resources, teams can quickly adjust spending according to service needs.
- Improved Flexibility:- It’s easier to begin the implementation of an app serverless than it is with traditional methods. Because of that, going serverless means you can innovate faster as well, It’s also easier to pivot in situations where you need to restructure.
- Pay-as-you-go Model:- It means that the consumers are only going to be charged the number of times their piece of code runs on a serverless service.
Let’s understand how serverless computing can have an impact on business growth.
- With Serverless computing architecture, enterprises can enhance scalability, enable pay-per-use capabilities and lower costs.
- Serverless computing has capabilities to eliminate infrastructure management tasks, reduce operating system maintenance costs, and encourage capacity provisioning and patching. Besides, the rising focus of companies towards serverless infrastructure is likely to offer lucrative opportunities in the market.
- Help improve operations by decreasing downtime and increasing overall efficiency saving them time and money in the process.
- Serverless computing will allow your enterprise to embrace digital transformation and optimize the opportunities created by the modernization of the application and infrastructure stack that will usher in new modes of automation, management, DevOps, and security.
Conclusion:
To sum up, Serverless Computing is the future of cloud computing. It provides the companies with the capability to be more agile, cost-effective and increase their overall operational efficiency. Serverless computing could be one of the most exciting developments of the 21st century. For those looking to build event-based apps quickly and efficiently, serverless computing is the way to conserve resources, increase efficiency, and boost productivity.
If this is the need of the hour for your business, we are here to help! Write to us at info@ankercloud.com and we will get back to you!
Migration of Servers from Digital Ocean to AWS
Hello, When starting the process of migrating servers from Digital Ocean to AWS. I choose AWS Application Migration Service after carefully reading the documentation to decide which AWS service to use for the migration.
Why I Selected the AWS Application Migration Service:
AWS Application Migration Service (MGN) is a highly automated lift-and-shift (rehost) solution that simplifies, expedites, and reduces the cost of migrating applications to AWS. It enables companies to lift-and-shift a large number of physical, virtual, or cloud servers without compatibility issues, performance disruption, or long cutover windows.
As per the Requirement of customers they want to clone their complete server as it is on AWS, so as MGN gives us Lift and Shift of everything we have on our servers I decide to go with it.
Moving to the Steps of Migration:
The Servers which are in the digital ocean they all are in the public subnet, so I have asked for the access of all the servers which I want to migrate. (If you don’t have access of source servers then share the replication agent download and installation link with your client)
1. Creating User:
I. As the First step you have to Login to AWS console-> go to the IAM-> create new user: MGNUSER->and add the permissions -> AWSApplicationMigrationAgentPolicy -> click create user
II. Then generate Access keys and Secret keys for the MGNUSER (we need these keys for creating source server in MGN.
2. Download and Installation of Replication Agent on Source
I. Navigate to Application Migration Console-> Go to Source Server-> Add server-> Select your OS type-> Enter Your MGNUSER Access key and Secret Key.

I. Then copy the replication download link and run it on source server.
II. Once downloaded, copy the replication installation agent link and run it on your source server.
III. It will take some time for the command line to appear on your source server (depending typically on network bandwidth and network connectivity between Digital Ocean and AWS). Keep your terminal open throughout this operation.
IV. Once the Process completed on your source server you can close your source terminal.
V. Note: The AWS Application Migration service did not support Ubuntu version 21
1. Configuration on AWS
I. Go to Application Migration Service in AWS It is possible to observe that the new server has been built and is currently synchronizing (again, it is not required that you remain logged into your AWS account and continuously monitor the developments).
II. The continuing Synchronous procedure will not be impacted by your network connectivity loss or logging out of your AWS account.
III. The Synchronous process will be completed and marked as being ready for testing (the Synchronous process takes hours to complete depending on the size of data on source).
2. Launch Test Instance
I. Select your server-> go to the Launch Configuration-> mention the Network Configurations-> size of instance-> its VPC-> subnet
II. Click on Launch Test instance

III. It will create the job Id which will track the complete process.

IV. Once the EC2 instance launched successfully you can see that instance in your console.
V. Test the Instance. If everything is good then mark it as revert for cutover.
Note: During the process the changes which you are performing on source that is digital ocean server will not automatically add to the Launched EC2 instance.
Example:
If I have 10 files on sources at the time of launching the instance and the instance launched in AWS.
Now that I’ve made changes on the digital ocean server, I’ve added one file there, making a total of 11 files.
But that will not reflect on my EC2 for that updated changes we have to Revert the instance back to testing and then Launch the test instance using same Launch template.
VI. Once test done Mark it as Ready for cutover.
1. Launch Cutover instance
Points to be noted before cutover:
Make a plan for cutover it should be in non-business hours
Don’t shutdown your source server before finalizing the cutover.
I. Select the Launch Template configuration, do the configurations.
II. Select Launch cutover Instances
III. The cutover job will be created, See the job progress
IV. Once the Instance Successfully Launched.
Note: It will Terminate your already Launched test instance and will Launch new Instance.
V. Click on Finalize Cutover
VI. Now you successfully migrated the Server from Digital Ocean to AWS
VII. Terminate your Digital Ocean server and start using the newly Migrated AWS Server.
Conclusion
If you have any questions about moving a server from Digital Ocean to AWS, I hope this blog will help. Check your OS version before moving, keep tabs on source updates before cutting the switch, choose a schedule for the switch, and follow the instructions provided. I hope this will save you time, money, and risk.
Migration Readiness Assessment (MRA) Tool Overview
Are you ready for the Cloud?
A successful cloud migration begins with a detailed analysis of goals, business plans, and resources currently used, with the initial purpose of gaining a clear understanding of the starting point, discovering the gaps to be filled, and developing a strong business case. It is in this exact context that our Accelerated Cloud Exploration (ACE) program comes into play: as an Advanced AWS Consulting Partner, a team of specialists from Ankercloud guides our customers through a complete assessment phase covering all these points to make a well-informed decision on whether and how to move to the cloud.
Ankercloud’s expertise is combined with a variety of professional tools offered by AWS, starting with the Migration Readiness Assessment (MRA), which represents the first hands-on activity to embark on the Cloud Exploration journey. A customized roadmap is built to define the successive actions to be taken.
What is the MRA tool?
The Migration Readiness Assessment (MRA) tool is used to assess the customer’s strengths and weaknesses following the 6 areas of the AWS Cloud Adoption Framework: Business, Platform, People, Governance, Operations, and Security. It evaluates existing skills and set-up on a scale from 1 to 5, highlighting the readiness level and giving an overall score for each main focus area and related subtopics.
How does it work?
The process requires you to answer 80+ questions and is typically fulfilled in a 1-day workshop, but it can also take several days. Due to the difficulty of the topics and the depth of the analysis, it is important that the customer’s team and the experts from Ankercloud work well together to finish the tasks.
What is the outcome?
After data collection, Ankercloud generates a full report that includes charts, scores, and data visualization. Together with the customer, we can look at the results and determine which areas need more work and which are already ready to move to the cloud.
But the MRA workshop is just the beginning.
To finish the business case, the Total Cost of Ownership (TCO) must be calculated, and it may be necessary to evaluate how the on-premise resources are set up.
The AWS Migration Evaluator and Migration Portfolio Assessment are suitable tools to fulfill the missing analysis and guide our customers towards the completion of the ACE Program, ready for a PoC implementation. Based on the MRA results as well as the outcome of the other components of ACE, our customers have full visibility of the strengths and weaknesses of a potential migration to the cloud and can make an informed and confident decision if and how to migrate.
Further learning…
Are you willing to discover what the AWS cloud can offer but unsure what the best way to start is? Want to know more about MRA and the other tools available to complete the assessment phase, as well as our Accelerated Cloud Exploration program? Don’t hesitate to reach out to us, we will be more than happy to solve all your doubts and give support for getting started with AWS cloud technologies.
Getting started with an AWS data lake
What is Data Lake?
A data lake is a sizable, central repository that enables businesses to store and handle enormous volumes of unstructured, raw data in a variety of formats. Many sources, such as transnational systems, social media, sensors, and more, can contribute data to a data lake.
What is AWS Data Lake?
An organization can store and handle enormous amounts of raw, unstructured data in numerous formats in a data lake, a sizable, centralised repository. A data lake may contain data from many different sources, such as transactional systems, social media, sensors, and more.
Need of Data lake :-
You should construct an AWS Data lake immediately if you are experiencing any of the difficulties listed below.
1. Companies without a single source of data and too many data storage. having trouble obtaining info from several sources.
2. The cost of storing data is out of control and data volume is growing daily.
3. The way that data is organized varies greatly. Businesses, for instance, have data from logs, IoT devices, user audits, and image galleries.
4. Big data analytics using slow data.
This would make it obvious to you whether your company needs Amazon Data Lake or not.
Services Under in AWS DATA LAKE :-
A data lake can be created and managed using a number of capabilities and services provided by Amazon Web Services (AWS). Organizations can store all of their structured and unstructured data in a data lake, which is a central repository that works at any scale. Here are a few characteristics of the Amazon data lake :
Amazon S3 :- The main storage service for constructing data lakes is Amazon S3 (Simple Storage Service). It offers scalable object storage for data of any size and kind.
AWS Glue :- Data transfer between data stores is simple with the help of AWS Glue, a fully-managed extract, transform, and load (ETL) service. Additionally, it has the ability to automatically find and collect metadata related to your data.
AWS Lambda Functions:- In AWS Data Lake, Lambda functions can play a crucial role in automating and enhancing data processing workflows. Data Transformation : Lambda functions can be used to transform data as it is ingested into the Data Lake. Event Processing where Lambda will do automatic processing of data reducing the need for manual intervention.The pay as you go model will help in optimize the costs in Data Lake architecture and Lambda being serverless, can automatically scale up and down to varying workloads.
Amazon Athena :- Data in Amazon S3 may be analyzed using conventional SQL thanks to Amazon Athena, an interactive query service. As there is no infrastructure to set up, it is serverless.
Amazon EMR :- Processing massive volumes of data using distributed frameworks like Hadoop, Spark, and Presto is simple with Amazon EMR (Elastic MapReduce), a fully-managed service.
AWS Lake Formation :- You may quickly and securely create a data lake using the AWS Lake Creation service. Data transformation, data access controls, and categorization are just a few of the functions it offers.
AWS Glue DataBrew :- It’s simple to clean and normalize data for analysis using Amazon Glue DataBrew, a visual tool for data preparation.
Amazon Redshift :- A cloud data warehouse called Amazon Redshift makes it simple to analyze sizable amounts of structured data. It is compatible with additional AWS services like Amazon EMR and AWS Glue.
Amazon Kinesis :- The platform for streaming data on AWS is called Amazon Kinesis. You can use it to gather, process, and analyze streaming real-time data from a variety of sources.
Amazon QuickSight :- For your data lake, it is simple to generate visualisations and dashboards using Amazon QuickSight, a cloud-based business intelligence solution.
These are just a handful of the numerous Amazon data lake functionalities that are offered. They offer an extensive collection of tools for creating, maintaining, and analyzing data lakes at scale when used collectively.
Advantages of AWS Data Lakes :-
You can safely store, examine, and share massive volumes of data at scale with the fully managed service provided by AWS Data Lake. Using AWS Data Lake has a number of benefits, such as:
Scalability :- Petabytes of data may be handled by AWS Data Lake, which scales itself as your data increases.
Cost-effective :- It’s a cost-effective method for handling massive volumes of data because you only pay for the storage and computing resources you really use.
Security :- To protect your data, Amazon Data Lake offers a number of strong security features, including access control, auditing, and encryption both in transit and at rest.
Flexible :- You can choose the appropriate tool for the task by utilizing AWS Data Lake, which supports a number of data formats, including structured, semi-structured, and unstructured data.
Integrations :- You may utilize the ideal tool for the job with Amazon Data Lake since it supports a wide range of data formats, including structured, semi-structured, and unstructured data.
Analytics :- Many analytics tools, like Amazon Athena, Amazon EMR, and Amazon Redshift, are available through AWS Data Lake, making it simple to query and analyze your data.
Collaboration :- When working with coworkers and business partners, Amazon Data Lake makes it simple to securely share data with other users and applications.
AWS Data Lake Architecture :-
Organizations can store, manage, and analyze vast amounts of data from several sources using the scalable and secure AWS Data Lake data repository. The architecture of AWS Data Lake typically consists of the following components:

Data Sources :-
Data from several sources, including databases, applications, IoT devices, and social media platforms, can be ingested by AWS Data Lake. These data sources could be local or online.
Data Ingestion :-
AWS offers a number of services, including Amazon Kinesis, AWS Glue, and AWS Data Pipeline, for importing data into the Data Lake.
Data Storage :-
Amazon S3, Amazon EBS, and Amazon Glacier are just a few of the storage solutions that AWS Data Lake provides. With its limitless scalability, superior durability, and affordable pricing, Amazon S3 is the most widely used storage option.
Data Catalog :-
Users may find, comprehend, and manage the data that is stored in the Data Lake using the data catalogue that is offered by AWS Glue. Column names, table definitions, and other metadata are included in the data catalogue.
Data Processing :-
For processing data kept in the Data Lake, AWS offers a number of services like Amazon EMR, AWS Glue, and Amazon Athena. These services can be utilized for activities including data analysis, data cleansing, and data transformation.
Data Visualization :-
AWS offers a number of services for displaying data from the Data Lake, including Amazon QuickSight, which enables customers to build interactive dashboards and reports.
Security and Governance :-
For the protection of the privacy, accuracy, and accessibility of the data kept in the Data Lake, AWS offers a number of security and governance capabilities. Encryption, access management, and audit recording are some of these characteristics.
All things considered, the design of AWS Data Lake offers a highly scalable, safe, and economical option for storing and processing huge volumes of data.
limitations of AWS Data Lakes :-
AWS Data Lake has a lot of benefits, but there are a few potential drawbacks to take into account as well:
Complexity :- It can be difficult to set up and administer an Amazon Data Lake, especially if you are unfamiliar with the AWS ecosystem.
Cost :- While AWS Data Lake can be inexpensive, if you plan to store a lot of data or make a lot of queries, this cost-effectiveness may not last.
Expertise :- You might need to have knowledge of data engineering, data architecture, and data analytics to make the most of AWS Data Lake.
Integration :- While many Amazon services are compatible with AWS Data Lake, not all third-party programs or data sources may be compatible with it.
Latency :- There can be some latency while accessing and searching your data, depending on how you configure your AWS Data Lake.
Maintenance :- Amazon Data Lake needs regular maintenance, just like any other IT system, to guarantee optimum performance and security. It may take a lot of time and resources to do this.
When deciding whether to use AWS Data Lake for your particular use case, it is crucial to balance these potential drawbacks with the advantages of doing so.
Conclusion :-
In general, AWS data lake offers a wide range of advantages, such as streamlined data administration, enhanced data quality and accessibility, accelerated time to insights, and cost savings. But setting up and maintaining an AWS data lake requires knowledge of data management and AWS services, so it’s crucial to carefully plan and design the architecture to make sure it satisfies the organization’s unique requirements.
.png)
Smart Risk Assessment: Bitech’s AI-Driven Solution for Property Insurance

Streamlining CI/CD: A Seamless Journey from Bitbucket to Elastic Beanstalk with AWS CodePipeline
.png)
Setting Up Google Cloud Account and Migrating Critical Applications for Rakuten India

Migration to Cloud and Setting Up of Analytics along With Managed Services
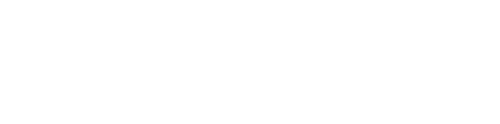
gocomo Migrates Social Data Platform to AWS for Performance and Scalability with Ankercloud
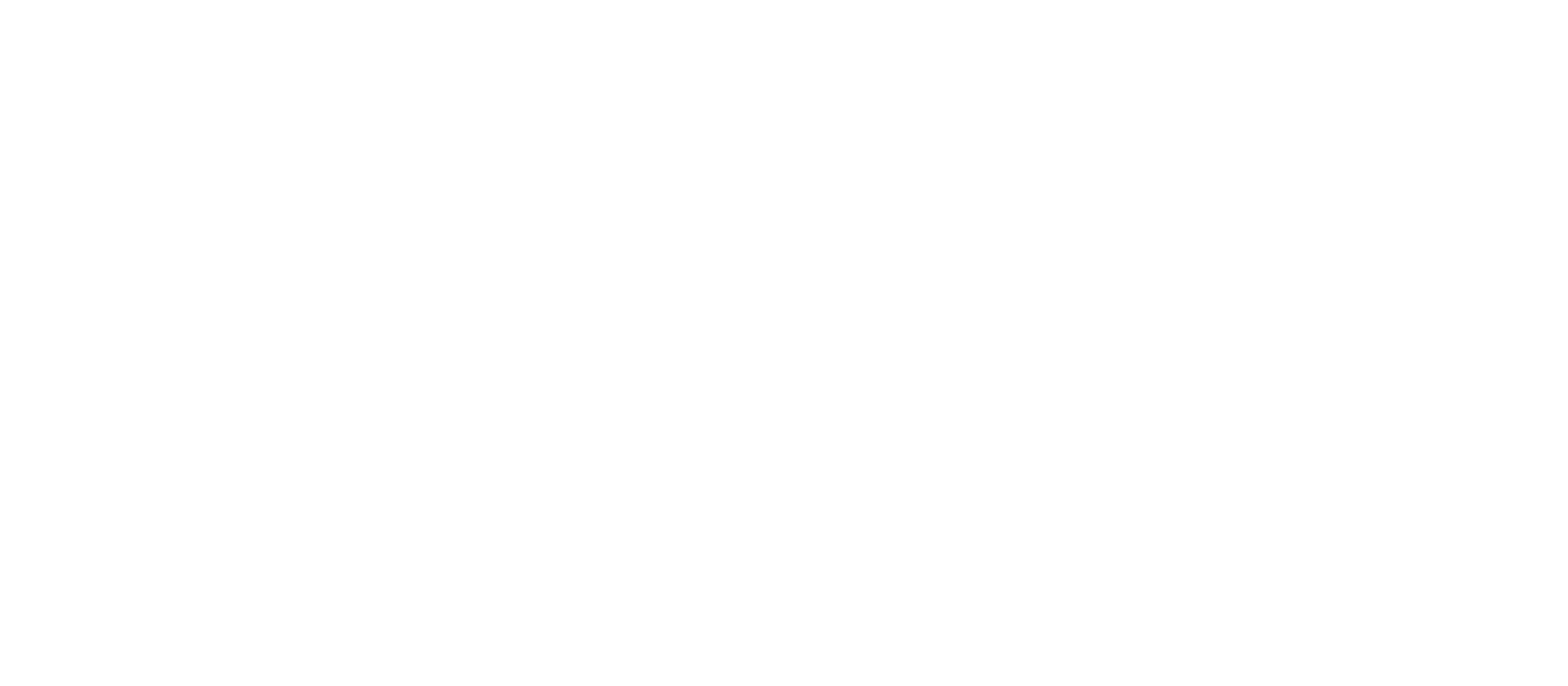
Benchmarking AWS performance to run environmental simulations over Belgium

Achieving Cost Optimization, Security, and Compliance: Ankercloud's AWS CloudOps Solutions for Federmeister
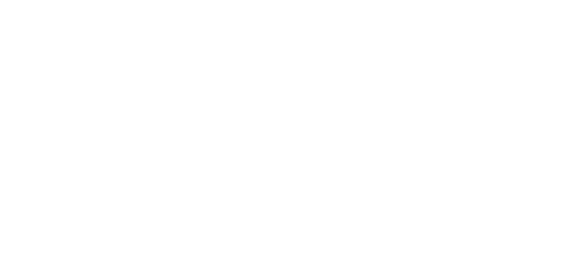
Model development for Image Object Classification and OCR analysis for mining industry
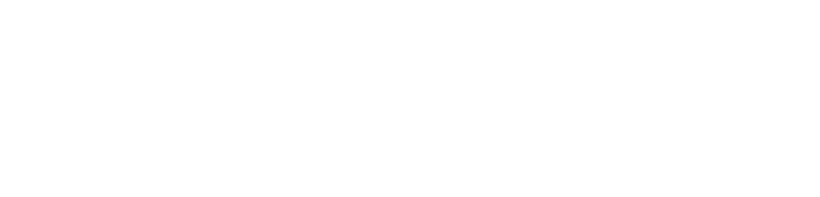
Replication of On-premise Infrastructure into AWS Cloud on Docker Swarm platform
Please Type Other Keywords
The Ankercloud Team loves to listen
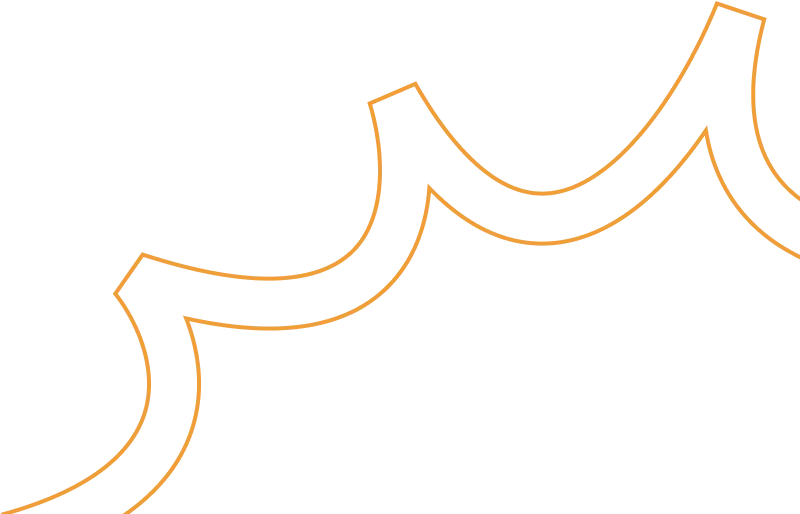
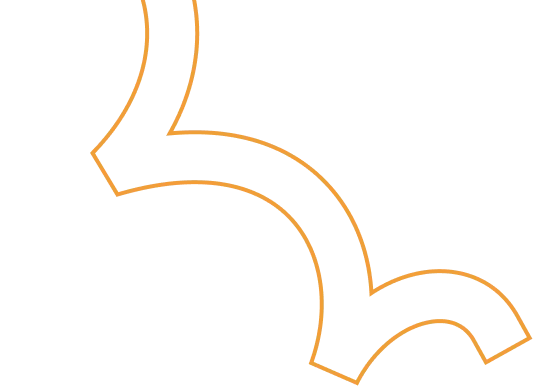